|
Rob Deardon Person1 #679869 Associate Professor in the Department of Production Animal Health in the Faculty of Veterinary Medicine and the Department of Mathematics and Statistics in the Faculty of Science at the University of Calgary. | 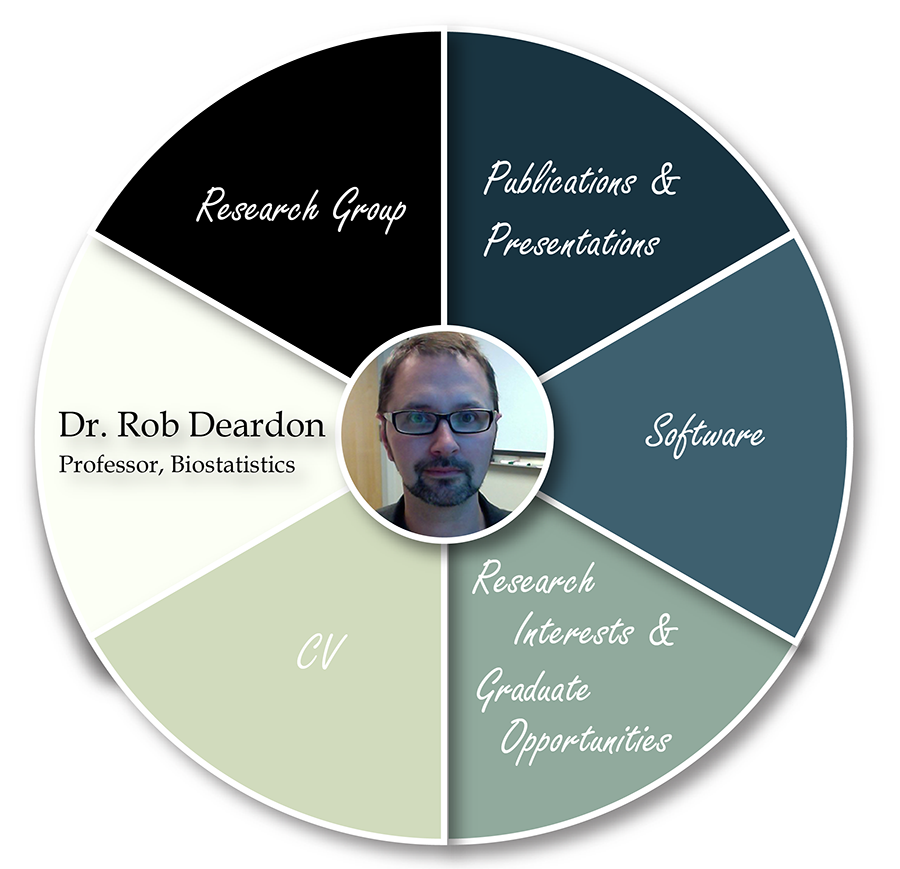
Current Research Interests1. STATISTICAL MODELING OF INFECTIOUS DISEASE - Diseases of humans, animals and plants
- Spatial systems
- Network-based systems
- Measurement error & latent information
- Robustness to assumptions
- Computational methodologies (see Bayesian & Computational Statistics below)
- Study design
- Model comparison
- Model adequacy/goodness of fit
- Disease surveillance models
2. BAYESIAN AND COMPUTATIONAL STATISTICS - Markov chain Monte Carlo methods (MCMC)
- Approximate Bayesian computation (ABC)
- Gaussian process emulation
- Machine learning-based approximate inference
- Importance sampling and sequential Monte Carlo (SMC)
3. ECOLOGICAL AND ENVIRONMENTAL MODELING - Spatial and spatiotemporal models (e.g. disease mapping)
- Invasive species models (e.g. Ash borer, Pine beetle, Giant hogweed)
- Fire spread models
- Animal movement models
4. EXPERIMENTAL DESIGN - Bayesian experimental design
- Crop trials (e.g. dealing with inter-plot interference in experiments on crop diseases)
- Spatial design for experiments used to ascertain infectious disease dynamics
- Response surface methodology and optimal design
5. STATISTICAL & MACHINE LEARNING - Random forests & ensemble methods
- Networks and deep learning
- High dimensional model selection (e.g. gene selection)
- Predictive modelling and classification
6. OTHER TOPICS - Bayesian clinical trials
- Network meta-analyses
- Discrete choice experiments
Tags: Robert Deardon |
+Citations (4) - CitationsAdd new citationList by: CiterankMapLink[2] A Framework for Incorporating Behavioural Change into Individual-Level Spatial Epidemic Models
Author: Madeline A. Ward, Rob Deardon, Lorna E. Deeth Publication date: 1 August 2023 Publication info: arXiv:2308.00815v1 [stat.ME] Cited by: David Price 10:29 PM 16 November 2023 GMT Citerank: (2) 715386Madeline WardMadeline A. Ward is a PHD student in Biostatistics in the Department of Mathematics and Statistics at the University of Calgary.10019D3ABAB, 715387SMMEID – Publications144B5ACA0 URL: DOI: https://doi.org/10.48550/arXiv.2308.00815
| Excerpt / Summary [arXiv, 1 August 2023]
During epidemics, people will often modify their behaviour patterns over time in response to changes in their perceived risk of spreading or contracting the disease. This can substantially impact the trajectory of the epidemic. However, most infectious disease models assume stable population behaviour due to the challenges of modelling these changes. We present a flexible new class of models, called behavioural change individual-level models (BC-ILMs), that incorporate both individual-level covariate information and a data-driven behavioural change effect. Focusing on spatial BC-ILMs, we consider four "alarm" functions to model the effect of behavioural change as a function of infection prevalence over time. We show how these models can be estimated in a simulation setting. We investigate the impact of misspecifying the alarm function when fitting a BC-ILM, and find that if behavioural change is present in a population, using an incorrect alarm function will still result in an improvement in posterior predictive performance over a model that assumes stable population behaviour. We also find that using spike and slab priors on alarm function parameters is a simple and effective method to determine whether a behavioural change effect is present in a population. Finally, we show results from fitting spatial BC-ILMs to data from the 2001 U.K. foot and mouth disease epidemic. |
Link[3] Bayesian modeling of dynamic behavioral change during an epidemic
Author: Caitlin Ward, Rob Deardon, Alexandra M. Schmidt Publication date: 11 August 2023 Publication info: Infectious Disease Modelling, Volume 8, Issue 4,
2023, Pages 947-963, ISSN 2468-0427, Cited by: David Price 10:36 PM 16 November 2023 GMT Citerank: (1) 715387SMMEID – Publications144B5ACA0 URL: DOI: https://doi.org/10.1016/j.idm.2023.08.002
| Excerpt / Summary [Infectious Disease Modelling, 11 August 2023]
For many infectious disease outbreaks, the at-risk population changes their behavior in response to the outbreak severity, causing the transmission dynamics to change in real-time. Behavioral change is often ignored in epidemic modeling efforts, making these models less useful than they could be. We address this by introducing a novel class of data-driven epidemic models which characterize and accurately estimate behavioral change. Our proposed model allows time-varying transmission to be captured by the level of “alarm” in the population, with alarm specified as a function of the past epidemic trajectory. We investigate the estimability of the population alarm across a wide range of scenarios, applying both parametric functions and non-parametric functions using splines and Gaussian processes. The model is set in the data-augmented Bayesian framework to allow estimation on partially observed epidemic data. The benefit and utility of the proposed approach is illustrated through applications to data from real epidemics. |
Link[4] Charting a future for emerging infectious disease modelling in Canada
Author: Mark A. Lewis, Patrick Brown, Caroline Colijn, Laura Cowen, Christopher Cotton, Troy Day, Rob Deardon, David Earn, Deirdre Haskell, Jane Heffernan, Patrick Leighton, Kumar Murty, Sarah Otto, Ellen Rafferty, Carolyn Hughes Tuohy, Jianhong Wu, Huaiping Zhu Publication date: 26 April 2023 Cited by: David Price 10:20 AM 15 December 2023 GMT
Citerank: (22) 679703EIDM?The Emerging Infectious Diseases Modelling Initiative (EIDM) – by the Public Health Agency of Canada and NSERC – aims to establish multi-disciplinary network(s) of specialists across the country in modelling infectious diseases to be applied to public needs associated with emerging infectious diseases and pandemics such as COVID-19. [1]7F1CEB7, 679761Caroline ColijnDr. Caroline Colijn works at the interface of mathematics, evolution, infection and public health, and leads the MAGPIE research group. She joined SFU's Mathematics Department in 2018 as a Canada 150 Research Chair in Mathematics for Infection, Evolution and Public Health. She has broad interests in applications of mathematics to questions in evolution and public health, and was a founding member of Imperial College London's Centre for the Mathematics of Precision Healthcare.10019D3ABAB, 679769Christopher CottonChristopher Cotton is a Professor of Economics at Queen’s University where he holds the Jarislowsky-Deutsch Chair in Economic & Financial Policy.10019D3ABAB, 679776David EarnProfessor of Mathematics and Faculty of Science Research Chair in Mathematical Epidemiology at McMaster University.10019D3ABAB, 679797Huaiping ZhuProfessor of mathematics at the Department of Mathematics and Statistics at York University, a York Research Chair (YRC Tier I) in Applied Mathematics, the Director of the Laboratory of Mathematical Parallel Systems at the York University (LAMPS), the Director of the Canadian Centre for Diseases Modelling (CCDM) and the Director of the One Health Modelling Network for Emerging Infections (OMNI-RÉUNIS). 10019D3ABAB, 679806Jane HeffernanJane Heffernan is a professor of infectious disease modelling in the Mathematics & Statistics Department at York University. She is a co-director of the Canadian Centre for Disease Modelling, and she leads national and international networks in mathematical immunology and the modelling of waning and boosting immunity.10019D3ABAB, 679812Jianhong WuProfessor Jianhong Wu is a University Distinguished Research Professor and Senior Canada Research Chair in industrial and applied mathematics at York University. He is also the NSERC Industrial Research Chair in vaccine mathematics, modelling, and manufacturing. 10019D3ABAB, 679826Laura CowenAssociate Professor in the Department of Mathematics and Statistics at the University of Victoria.10019D3ABAB, 679842Mark LewisProfessor Mark Lewis, Kennedy Chair in Mathematical Biology at the University of Victoria and Emeritus Professor at the University of Alberta.10019D3ABAB, 679858Patrick BrownAssociate Professor in the Centre for Global Health Research at St. Michael’s Hospital, and in the Department of Statistical Sciences at the University of Toronto.10019D3ABAB, 679859Patrick LeightonPatrick Leighton is a Professor of Epidemiology and Public Health at the Faculty of Veterinary Medicine, University of Montreal, and an active member of the Epidemiology of Zoonoses and Public Health Research Group (GREZOSP) and the Centre for Public Health Research (CReSP). 10019D3ABAB, 679875Sarah OttoProfessor in Zoology. Theoretical biologist, Canada Research Chair in Theoretical and Experimental Evolution, and Killam Professor at the University of British Columbia.10019D3ABAB, 679890Troy DayTroy Day is a Professor and the Associate Head of the Department of Mathematics and Statistics at Queen’s University. He is an applied mathematician whose research focuses on dynamical systems, optimization, and game theory, applied to models of infectious disease dynamics and evolutionary biology.10019D3ABAB, 679893Kumar MurtyProfessor Kumar Murty is in the Department of Mathematics at the University of Toronto. His research fields are Analytic Number Theory, Algebraic Number Theory, Arithmetic Algebraic Geometry and Information Security. He is the founder of the GANITA lab, co-founder of Prata Technologies and PerfectCloud. His interest in mathematics ranges from the pure study of the subject to its applications in data and information security.10019D3ABAB, 686724Ellen RaffertyDr. Ellen Rafferty has a Master of Public Health and a PhD in epidemiology and health economics from the University of Saskatchewan. Dr. Rafferty’s research focuses on the epidemiologic and economic impact of public health policies, such as estimating the cost-effectiveness of immunization programs. She is interested in the incorporation of economics into immunization decision-making, and to that aim has worked with a variety of provincial and national organizations.10019D3ABAB, 701020CANMOD – PublicationsPublications by CANMOD Members144B5ACA0, 701037MfPH – Publications144B5ACA0, 701071OSN – Publications144B5ACA0, 701222OMNI – Publications144B5ACA0, 704045Covid-19859FDEF6, 714608Charting a FutureCharting a Future for Emerging Infectious Disease Modelling in Canada – April 2023 [1] 2794CAE1, 715387SMMEID – Publications144B5ACA0 URL:
| Excerpt / Summary We propose an independent institute of emerging infectious disease modellers and policy experts, with an academic core, capable of renewing itself as needed. This institute will combine science and knowledge translation to inform decision-makers at all levels of government and ensure the highest level of preparedness (and readiness) for the next public health emergency. The Public Health Modelling Institute will provide cost-effective, science-based modelling for public policymakers in an easily visualizable, integrated framework, which can respond in an agile manner to changing needs, questions, and data. To be effective, the Institute must link to modelling groups within government, who are best able to pose questions and convey results for use by public policymakers. |
|
|