|
Jonathan Dushoff Person1 #679814 Professor in the Department Of Biology at McMaster University. | 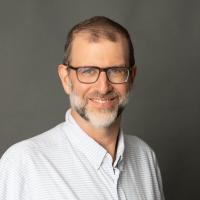
- Jonathan is a theoretical biologist with a broad interest in computational and statistical approaches to practical biological questions with a particular interest in dynamical questions (how systems are shaped at large scales by the way that they change at small scales).
- His current research is mostly focused on infectious diseases of humans.
|
+Citations (11) - CitationsAdd new citationList by: CiterankMapLink[3] Immune boosting bridges leaky and polarized vaccination models
Author: Sang Woo Park, Michael Li, C. Jessica E. Metcalf, Bryan T. Grenfell, Jonathan Dushoff Publication date: 30 July 2023 Publication info: medRxiv 2023.07.14.23292670 Cited by: David Price 8:42 PM 16 November 2023 GMT Citerank: (2) 701020CANMOD – PublicationsPublications by CANMOD Members144B5ACA0, 704041Vaccination859FDEF6 URL: DOI: https://doi.org/10.1101/2023.07.14.23292670
| Excerpt / Summary [medRxiv, 30 July 2023]
Two different epidemiological models of vaccination are commonly used in dynamical modeling studies. The leaky vaccination model assumes that all vaccinated individuals experience a reduced force of infection by the same amount. The polarized vaccination model assumes that some fraction of vaccinated individuals are completely protected, while the remaining fraction remains completely susceptible; this seemingly extreme assumption causes the polarized model to always predict lower final epidemic size than the leaky model under the same vaccine efficacy. However, the leaky model also makes an implicit, unrealistic assumption: vaccinated individuals who are exposed to infection but not infected remain just as susceptible as they were prior to exposures (i.e., independent of previous exposures). To resolve the independence assumption, we introduce an immune boosting mechanism, through which vaccinated, yet susceptible, individuals can gain protection without developing a transmissible infection. The boosting model further predicts identical epidemic dynamics as the polarized vaccination model, thereby bridging the differences between two models. We further develop a generalized vaccination model to explore how the assumptions of immunity affect epidemic dynamics and estimates of vaccine effectiveness. |
Link[4] Evaluating undercounts in epidemics: response to Maruotti et al. 2022
Author: Michael Li, Jonathan Dushoff, David J. D. Earn, Benjamin M. Bolker Publication date: 22 September 2022 Publication info: arXiv:2209.11334 [q-bio.PE] Cited by: David Price 12:16 PM 25 November 2023 GMT Citerank: (6) 679758Benjamin BolkerI’m a professor in the departments of Mathematics & Statistics and of Biology at McMaster University, and currently Director of the School of Computational Science and Engineering and Acting Associate Chair (Graduate) for Mathematics.10019D3ABAB, 679776David EarnProfessor of Mathematics and Faculty of Science Research Chair in Mathematical Epidemiology at McMaster University.10019D3ABAB, 685445Michael WZ LiMichael Li is Senior Scientist in the Public Health Risk Science Division (PHRS) of the Public Health Agency of Canada (PHAC) and a Research Associate at the South African Centre for Epidemiological Modelling and Analysis (SACEMA).10019D3ABAB, 701020CANMOD – PublicationsPublications by CANMOD Members144B5ACA0, 701037MfPH – Publications144B5ACA0, 715667mpox859FDEF6 URL: DOI: https://doi.org/10.48550/arXiv.2209.11334
| Excerpt / Summary [arXiv, 22 September 2022]
Maruotti et al. 2022 used a mark-recapture approach to estimate bounds on the true number of monkeypox infections in various countries. These approaches are fundamentally flawed; it is impossible to estimate undercounting based solely on a single stream of reported cases. Simulations based on a Richards curve for cumulative incidence show that, for reasonable epidemic parameters, the proposed methods estimate bounds on the ascertainment ratio of ≈0.2−0.5 roughly independently of the true ascertainment ratio. These methods should not be used. |
Link[5] How time-scale differences in asymptomatic and symptomatic transmission shape SARS-CoV-2 outbreak dynamics
Author: Jeremy D. Harris, Sang Woo Park, Jonathan Dushoff, Joshua S. Weitz Publication date: 25 January 2023 Publication info: Epidemics, Volume 42, March 2023, 100664, ISSN 1755-4365, Cited by: David Price 11:10 PM 25 November 2023 GMT Citerank: (2) 701020CANMOD – PublicationsPublications by CANMOD Members144B5ACA0, 704045Covid-19859FDEF6 URL: DOI: https://doi.org/10.1016/j.epidem.2022.100664
| Excerpt / Summary [Epidemics, 25 January 2023]
Asymptomatic and symptomatic SARS-CoV-2 infections can have different characteristic time scales of transmission. These time-scale differences can shape outbreak dynamics as well as bias population-level estimates of epidemic strength, speed, and controllability. For example, prior work focusing on the initial exponential growth phase of an outbreak found that larger time scales for asymptomatic vs. symptomatic transmission can lead to under-estimates of the basic reproduction number as inferred from epidemic case data. Building upon this work, we use a series of nonlinear epidemic models to explore how differences in asymptomatic and symptomatic transmission time scales can lead to changes in the realized proportion of asymptomatic transmission throughout an epidemic. First, we find that when asymptomatic transmission time scales are longer than symptomatic transmission time scales, then the effective proportion of asymptomatic transmission increases as total incidence decreases. Moreover, these time-scale-driven impacts on epidemic dynamics are enhanced when infection status is correlated between infector and infectee pairs (e.g., due to dose-dependent impacts on symptoms). Next we apply these findings to understand the impact of time-scale differences on populations with age-dependent assortative mixing and in which the probability of having a symptomatic infection increases with age. We show that if asymptomatic generation intervals are longer than corresponding symptomatic generation intervals, then correlations between age and symptoms lead to a decrease in the age of infection during periods of epidemic decline (whether due to susceptible depletion or intervention). Altogether, these results demonstrate the need to explore the role of time-scale differences in transmission dynamics alongside behavioral changes to explain outbreak features both at early stages (e.g., in estimating the basic reproduction number) and throughout an epidemic (e.g., in connecting shifts in the age of infection to periods of changing incidence). |
Link[6] The need for linked genomic surveillance of SARS-CoV-2
Author: Caroline Colijn, David JD Earn, Jonathan Dushoff, Nicholas H Ogden, Michael Li, Natalie Knox, Gary Van Domselaar, Kristyn Franklin, Gordon Jolly, Sarah P Otto Publication date: 6 April 2022 Publication info: Can Commun Dis Rep. 2022 Apr 6; 48(4): 131–139, PMCID: PMC9017802PMID: 35480703 Cited by: David Price 10:34 PM 29 November 2023 GMT
Citerank: (11) 679761Caroline ColijnDr. Caroline Colijn works at the interface of mathematics, evolution, infection and public health, and leads the MAGPIE research group. She joined SFU's Mathematics Department in 2018 as a Canada 150 Research Chair in Mathematics for Infection, Evolution and Public Health. She has broad interests in applications of mathematics to questions in evolution and public health, and was a founding member of Imperial College London's Centre for the Mathematics of Precision Healthcare.10019D3ABAB, 679875Sarah OttoProfessor in Zoology. Theoretical biologist, Canada Research Chair in Theoretical and Experimental Evolution, and Killam Professor at the University of British Columbia.10019D3ABAB, 685445Michael WZ LiMichael Li is Senior Scientist in the Public Health Risk Science Division (PHRS) of the Public Health Agency of Canada (PHAC) and a Research Associate at the South African Centre for Epidemiological Modelling and Analysis (SACEMA).10019D3ABAB, 701020CANMOD – PublicationsPublications by CANMOD Members144B5ACA0, 701023GenomicsWhile virus genomes can describe the global context of introductions and origins of local clusters of cases, CANMOD will focus on building methods for characterizing and modelling local transmission once it is established, and for surveillance for viral determinants of increased fitness and of enhanced risk of spillover, virulence and transmission.859FDEF6, 701037MfPH – Publications144B5ACA0, 704045Covid-19859FDEF6, 707634Gary Van DomselaarDr. Gary Van Domselaar, PhD (University of Alberta, 2003) is the Chief of the Bioinformatics Laboratory at the National Microbiology Laboratory in Winnipeg Canada, and Adjunct Professor in the Department of Medical Microbiology at the University of Manitoba.10019D3ABAB, 708734Genomics859FDEF6, 715277Covid-19Covid-19 » Relevance » Genomics10000FFFACD, 715329Nick OgdenNicholas Ogden is a senior research scientist and Director of the Public Health Risk Sciences Division within the National Microbiology Laboratory at the Public Health Agency of Canada.10019D3ABAB URL: DOI: https://doi.org/10.14745/ccdr.v48i04a03
| Excerpt / Summary [Canada Communicable Disease Report, 6 April 2022]
Genomic surveillance during the coronavirus disease 2019 (COVID-19) pandemic has been key to the timely identification of virus variants with important public health consequences, such as variants that can transmit among and cause severe disease in both vaccinated or recovered individuals. The rapid emergence of the Omicron variant highlighted the speed with which the extent of a threat must be assessed. Rapid sequencing and public health institutions’ openness to sharing sequence data internationally give an unprecedented opportunity to do this; however, assessing the epidemiological and clinical properties of any new variant remains challenging. Here we highlight a “band of four” key data sources that can help to detect viral variants that threaten COVID-19 management: 1) genetic (virus sequence) data; 2) epidemiological and geographic data; 3) clinical and demographic data; and 4) immunization data. We emphasize the benefits that can be achieved by linking data from these sources and by combining data from these sources with virus sequence data. The considerable challenges of making genomic data available and linked with virus and patient attributes must be balanced against major consequences of not doing so, especially if new variants of concern emerge and spread without timely detection and action. |
Link[7] Inferring the differences in incubation-period and generation-interval distributions of the Delta and Omicron variants of SARS-CoV-2
Author: Sang Woo Park, Kaiyuan Sun, Sam Abbott, Ron Sender, Yinon M. Bar-on, Joshua S. Weitz, Sebastian Funk, Bryan T. Grenfell, Jantien A. Backer, Jacco Wallinga, Cecile Viboud, Jonathan Dushoff Publication date: 22 May 2023 Publication info: PNAS, 22 May 2023, 120 (22) e2221887120 Cited by: David Price 4:45 PM 11 December 2023 GMT Citerank: (2) 701020CANMOD – PublicationsPublications by CANMOD Members144B5ACA0, 704045Covid-19859FDEF6 URL: DOI: https://doi.org/10.1073/pnas.2221887120
| Excerpt / Summary [PNAS, 22 May 2023]
Estimating the differences in the incubation-period, serial-interval, and generation-interval distributions of SARS-CoV-2 variants is critical to understanding their transmission. However, the impact of epidemic dynamics is often neglected in estimating the timing of infection—for example, when an epidemic is growing exponentially, a cohort of infected individuals who developed symptoms at the same time are more likely to have been infected recently. Here, we reanalyze incubation-period and serial-interval data describing transmissions of the Delta and Omicron variants from the Netherlands at the end of December 2021. Previous analysis of the same dataset reported shorter mean observed incubation period (3.2 d vs. 4.4 d) and serial interval (3.5 d vs. 4.1 d) for the Omicron variant, but the number of infections caused by the Delta variant decreased during this period as the number of Omicron infections increased. When we account for growth-rate differences of two variants during the study period, we estimate similar mean incubation periods (3.8 to 4.5 d) for both variants but a shorter mean generation interval for the Omicron variant (3.0 d; 95% CI: 2.7 to 3.2 d) than for the Delta variant (3.8 d; 95% CI: 3.7 to 4.0 d). The differences in estimated generation intervals may be driven by the “network effect”—higher effective transmissibility of the Omicron variant can cause faster susceptible depletion among contact networks, which in turn prevents late transmission (therefore shortening realized generation intervals). Using up-to-date generation-interval distributions is critical to accurately estimating the reproduction advantage of the Omicron variant. |
Link[8] SARS-CoV-2 Incubation Period during Omicron BA.5–Dominant Period, Japan
Author: Hao-Yuan Cheng, Andrei R. Akhmetzhanov, Jonathan Dushoff Publication date: 1 January 2024 Publication info: Emerging Infectious Diseases. 2024;30(1):206-207, Cited by: David Price 9:44 PM 10 January 2024 GMT Citerank: (2) 701020CANMOD – PublicationsPublications by CANMOD Members144B5ACA0, 704045Covid-19859FDEF6 URL: DOI: https://doi.org/10.3201/eid3001.230208
| Excerpt / Summary [Emerging Infectious Diseases, 1 January 2024]
To the Editor: Ogata and Tanaka (1) estimated the mean incubation period was 2.9 (95% CI 2.6–3.2) days for SARS-CoV-2 strain Omicron BA.1 and 2.6 (95% CI 2.5–2.8) days for Omicron BA.5 during the Omicron-dominant period in Japan. Their earlier study reported a similar mean incubation period of 3.1 days for BA.1 (2). Their findings were derived from data collected through contact tracing efforts in Ibaraki Prefecture, Japan, which provided high accuracy in determining exposure time windows.
A potential concern is that their study only included cases that had a single exposure event and a 1-day exposure window. Although this concern was recognized by the authors as a study limitation, we emphasize that those criteria might bias results downward, especially when the disease is widespread. Persons that had longer incubation periods might have more opportunity for contacts or multiple exposure dates; thus, those with shorter incubation periods would be favored for inclusion. A more flexible case-selection approach might reduce bias, even though this approach would require methods to address uncertainty in actual infection timing.
In Taiwan, we collected data from the first 100 local symptomatic cases during the BA.1–dominant period (December 25, 2021–January 18, 2022), which were characterized by intensive case finding and contact tracing (A. Akhmetzhanov et al., unpub. data, https://doi.org/10.110...). Among 69 cases with an identified exposure, only 4 had a 1-day exposure window. Using more comprehensive exposure windows, the estimated mean incubation period in Taiwan was 3.5 (95% CI 3.1–4.0) days, longer than Tanaka et al.’s estimates (1,2) but similar to estimates of 3.5 days from Italy (data collected during January 2022) (3) and South Korea (data collected during November–December 2021) (4) and estimates from a systematic review (3.6 days) (5). The estimates from Japan (2) appear to be the shortest periods reported across previously reviewed studies (5). |
Link[9] Incubation-period estimates of Omicron (BA.1) variant from Taiwan, December 2021–January 2022, and its comparison to other SARS-CoV-2 variants: a statistical modeling, systematic search and meta-analysis
Author: Andrei R. Akhmetzhanov, Hao-Yuan Cheng, Jonathan Dushoff Publication date: 24 July 2023 Publication info: medRxiv 2023.07.20.23292983 Cited by: David Price 10:05 PM 10 January 2024 GMT Citerank: (2) 701020CANMOD – PublicationsPublications by CANMOD Members144B5ACA0, 704045Covid-19859FDEF6 URL: DOI: https://doi.org/10.1101/2023.07.20.23292983
| Excerpt / Summary [medRxiv, 24 July 2023]
Background: The ongoing COVID-19 pandemic has seen several variants of concern, including the Omicron (BA.1) variant which emerged in October 2021. Accurately estimating the incubation period of these variants is crucial for predicting disease spread and formulating effective public health strategies. However, existing estimates often conflict because of biases arising from the dynamic nature of epidemic growth and selective inclusion of cases. This study aims to accurately estimate of the Omicron (BA.1) variant incubation period based on data from Taiwan, where disease incidence remained low and contact tracing was comprehensive during the first months of the Omicron outbreak.
Methods: We reviewed 100 contact-tracing records for cases of the Omicron BA.1 variant reported between December 2021 and January 2022, and found enough information to analyze 70 of these. The incubation period distribution was estimated by fitting data on exposure and symptom onset within a Bayesian mixture model using gamma, Weibull, and lognormal distributions as candidates. Additionally, a systematic literature search was conducted to accumulate data for estimates of the incubation period for Omicron (BA.1/2, BA.4/5) subvariants, which was then used for meta-analysis and comparison.
Results: The mean incubation period was estimated at 3.5 days (95% credible interval: 3.1–4.0 days), with no clear differences when stratified by vaccination status or age. This estimate aligns closely with the pooled mean of 3.4 days (3.0–3.8 days) obtained from a meta-analysis of other published studies on Omicron subvariants.
Conclusions: The relatively shorter incubation period of the Omicron variant, as compared to previous SARS-CoV2 variants, implies its potential for rapid spread but also opens the possibility for individuals to voluntarily adopt shorter, more resource-efficient quarantine periods. Continual updates to incubation period estimates, utilizing data from comprehensive contact tracing, are crucial for effectively guiding these voluntary actions and adjusting high socio-economic cost interventions. |
Link[10] The probability of epidemic burnout in the stochastic SIR model with vital dynamics
Author: Todd L. Parsons, Benjamin M. Bolker, Jonathan Dushoff, David J. D. Earn Publication date: 26 January 2024 Publication info: PNAS, 121 (5) e2313708120 Cited by: David Price 1:10 AM 28 February 2024 GMT Citerank: (3) 679758Benjamin BolkerI’m a professor in the departments of Mathematics & Statistics and of Biology at McMaster University, and currently Director of the School of Computational Science and Engineering and Acting Associate Chair (Graduate) for Mathematics.10019D3ABAB, 679776David EarnProfessor of Mathematics and Faculty of Science Research Chair in Mathematical Epidemiology at McMaster University.10019D3ABAB, 701020CANMOD – PublicationsPublications by CANMOD Members144B5ACA0 URL: DOI: https://doi.org/10.1073/pnas.2313708120
| Excerpt / Summary [PNAS, 26 January 2024]
If a new pathogen causes a large epidemic, then it might “burn out” before causing a second epidemic. The burnout probability can be estimated from large numbers of computationally intensive simulations, but an easily computable formula for the burnout probability has never been found. Using a conceptually simple approach, we derive such a formula for the standard SIR epidemic model with vital dynamics (host births and deaths). With this formula, we show that the burnout probability is always smaller for diseases with longer infectious periods, but is bimodal with respect to transmissibility (the basic reproduction number). Our analysis shows that the persistence of typical human infectious diseases cannot be explained by births of new susceptibles, clarifying an important epidemiological puzzle… |
Link[11] Over a Century of Infectious Disease Surveillance in Canada: Introducing the Canadian Disease Incidence Dataset (CANDID)
Author: David J. D. Earn, Gabrielle MacKinnon, Samara Manzin, Michael Roswell, Steve Cygu, Chyunfung Shi, Benjamin M. Bolker, Jonathan Dushoff, Steven C. Walker Publication date: 20 December 2024 Publication info: medRxiv 2024.12.20.24319425 Cited by: David Price 0:17 AM 24 March 2025 GMT Citerank: (6) 679758Benjamin BolkerI’m a professor in the departments of Mathematics & Statistics and of Biology at McMaster University, and currently Director of the School of Computational Science and Engineering and Acting Associate Chair (Graduate) for Mathematics.10019D3ABAB, 679776David EarnProfessor of Mathematics and Faculty of Science Research Chair in Mathematical Epidemiology at McMaster University.10019D3ABAB, 699814Canadian Disease Incidence Dataset (CANDID)We are making Canada's historical infectious disease incidence and mortality data publicly and conveniently available. [3]161D8AECC, 699816CANMOD – DataWe are working to collect, digitize, and curate infectious disease data, and to make these data accessible to modellers and other stakeholders.161D8AECC, 701020CANMOD – PublicationsPublications by CANMOD Members144B5ACA0, 715290Steve WalkerSteve is the CANMOD Director of Data Science and a postdoctoral fellow in the Department of Mathematics and Statistics at McMaster University.10019D3ABAB URL: DOI: https://doi.org/10.1101/2024.12.20.24319425
| Excerpt / Summary [medRxiv, 20 December 2024]
Background: Canadian notifiable disease surveillance provides data on the incidence of communicable diseases, dating back to the late 19th century. The Public Health Agency of Canada offers summaries of these data (from 1924–2022) through an online portal. These summaries provide historical context for Canadian health researchers, but lack information on intra-annual and inter-provincial patterns. Sub-annual and sub-national data can be found in published documents, or requested from archives of government agencies, but are only available in typewritten or handwritten hard copies. We digitized and collated these data sources to create a resource for epidemiology and public health.
Methods: We manually entered data from scans of hard copies into spreadsheets resembling the originals, facilitating accurate transcription through easier cross-checking. We developed open-source pipelines to harmonize these spreadsheets into CSV files that blend data across sources.
Results: We assembled and processed 1,631,380 incidence values from 1903–2021. Focusing on sub-annual and sub-national data and removing redundancy yielded 934,009 weekly, monthly, or quarterly incidence values broken down by province/territory, containing 139 diseases. We give two examples of sub-annual and sub-national patterns: strong annual cycles of poliomyelitis that peaked simultaneously across provinces, and spatially heterogeneous resurgence of whooping cough in the 1990s.
Interpretation: Canada’s history of infectious disease surveillance has produced a detailed record of sub-annual and sub-national disease incidence patterns that remains largely unexplored. This important record is now available as the Canadian Disease Incidence Dataset (CANDID), hosted on a publicly accessible website along with the pipelines used to create it and scans of the original sources. |
|
|