|
David Buckeridge Person1 #679775 David is a Professor in the School of Population and Global Health at McGill University, where he directs the Surveillance Lab, an interdisciplinary group that develops, implements, and evaluates novel computational methods for population health surveillance. He is also the Chief Digital Health Officer at the McGill University Health Center where he directs strategy on digital transformation and analytics and he is an Associate Member with the Montreal Institute for Learning Algorithms (Mila). | 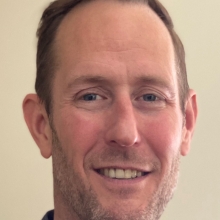
- His research and practice focus on the informatics of health surveillance and disease control and he holds a Canada Research Chair (Tier 1) in Health Informatics and Data Science. In the context of the COVID-19 pandemic, Dr Buckeridge provides regular projections of health system demand for the Canadian province of Quebec, is the Scientific lead for Data Management and Analytics for the Canadian Immunity Task Force, and is funded by the World Health Organization (WHO) to monitor global immunity to SARS-CoV-2. He is also a technical advisor to the WHO Epidemic Intelligence from Open Sources (EIOS) program on the application of artificial intelligence to global infectious disease surveillance. Dr Buckeridge has a MD from Queen's University, a MSc in Epidemiology from the University of Toronto, a PhD in Biomedical informatics from Stanford University, and is a Fellow of the Royal College of Physicians of Canada.
|
+Citations (6) - CitationsAdd new citationList by: CiterankMapLink[3] Applied artificial intelligence in healthcare: Listening to the winds of change in a post-COVID-19 world
Author: Arash Shaban-Nejad, Martin Michalowski, Robert L Davis, et al. Publication date: 25 November 2022 Publication info: Experimental Biology and Medicine, Volume 247, Issue 22 Cited by: David Price 9:04 PM 26 November 2023 GMT Citerank: (2) 701020CANMOD – PublicationsPublications by CANMOD Members144B5ACA0, 704019Artificial intelligence859FDEF6 URL: DOI: https://doi.org/10.1177/1535370222114040
| Excerpt / Summary [Experimental Biology and Medicine, 25 November 2022]
This editorial article aims to highlight advances in artificial intelligence (AI) technologies in five areas: Collaborative AI, Multimodal AI, Human-Centered AI, Equitable AI, and Ethical and Value-based AI in order to cope with future complex socioeconomic and public health issues. |
Link[4] Global SARS-CoV-2 seroprevalence from January 2020 to April 2022: A systematic review and meta-analysis of standardized population-based studies
Author: Isabel Bergeri, Mairead G. Whelan, Harriet Ware, et al. Unity Studies Collaborator Group - Lorenzo Subissi, Anthony Nardone, Hannah C. Lewis, Zihan Li,Xiaomeng Ma, Marta Valenciano, Brianna Cheng, Lubna Al Ariqi, Arash Rashidian, Joseph Okeibunor, Tasnim Azim, Pushpa Wijesinghe, Linh-Vi Le, Aisling Vaughan, Richard Pebody, Andrea Vicari, Tingting Yan, Mercedes Yanes-Lane, Christian Cao, David A. Clifton, Matthew P. Cheng, Jesse Papenburg, David Buckeridge, Niklas Bobrovitz, Rahul K. Arora, Maria D. Van Kerkhove Publication date: 10 November 2022 Publication info: PLoS Med 19(11): e1004107 Cited by: David Price 9:12 PM 26 November 2023 GMT Citerank: (3) 701020CANMOD – PublicationsPublications by CANMOD Members144B5ACA0, 704045Covid-19859FDEF6, 715376Serosurveillance859FDEF6 URL: DOI: https://doi.org/10.1371/journal.pmed.1004107
| Excerpt / Summary [PLoS Medicine, 10 November 2022]
Background: Our understanding of the global scale of Severe Acute Respiratory Syndrome Coronavirus 2 (SARS-CoV-2) infection remains incomplete: Routine surveillance data underestimate infection and cannot infer on population immunity; there is a predominance of asymptomatic infections, and uneven access to diagnostics. We meta-analyzed SARS-CoV-2 seroprevalence studies, standardized to those described in the World Health Organization’s Unity protocol (WHO Unity) for general population seroepidemiological studies, to estimate the extent of population infection and seropositivity to the virus 2 years into the pandemic.
Methods and findings: We conducted a systematic review and meta-analysis, searching MEDLINE, Embase, Web of Science, preprints, and grey literature for SARS-CoV-2 seroprevalence published between January 1, 2020 and May 20, 2022. The review protocol is registered with PROSPERO (CRD42020183634). We included general population cross-sectional and cohort studies meeting an assay quality threshold (90% sensitivity, 97% specificity; exceptions for humanitarian settings). We excluded studies with an unclear or closed population sample frame. Eligible studies—those aligned with the WHO Unity protocol—were extracted and critically appraised in duplicate, with risk of bias evaluated using a modified Joanna Briggs Institute checklist. We meta-analyzed seroprevalence by country and month, pooling to estimate regional and global seroprevalence over time; compared seroprevalence from infection to confirmed cases to estimate underascertainment; meta-analyzed differences in seroprevalence between demographic subgroups such as age and sex; and identified national factors associated with seroprevalence using meta-regression. We identified 513 full texts reporting 965 distinct seroprevalence studies (41% low- and middle-income countries [LMICs]) sampling 5,346,069 participants between January 2020 and April 2022, including 459 low/moderate risk of bias studies with national/subnational scope in further analysis. By September 2021, global SARS-CoV-2 seroprevalence from infection or vaccination was 59.2%, 95% CI [56.1% to 62.2%]. Overall seroprevalence rose steeply in 2021 due to infection in some regions (e.g., 26.6% [24.6 to 28.8] to 86.7% [84.6% to 88.5%] in Africa in December 2021) and vaccination and infection in others (e.g., 9.6% [8.3% to 11.0%] in June 2020 to 95.9% [92.6% to 97.8%] in December 2021, in European high-income countries [HICs]). After the emergence of Omicron in March 2022, infection-induced seroprevalence rose to 47.9% [41.0% to 54.9%] in Europe HIC and 33.7% [31.6% to 36.0%] in Americas HIC. In 2021 Quarter Three (July to September), median seroprevalence to cumulative incidence ratios ranged from around 2:1 in the Americas and Europe HICs to over 100:1 in Africa (LMICs). Children 0 to 9 years and adults 60+ were at lower risk of seropositivity than adults 20 to 29 (p < 0.001 and p = 0.005, respectively). In a multivariable model using prevaccination data, stringent public health and social measures were associated with lower seroprevalence (p = 0.02). The main limitations of our methodology include that some estimates were driven by certain countries or populations being overrepresented.
Conclusions: In this study, we observed that global seroprevalence has risen considerably over time and with regional variation; however, over one-third of the global population are seronegative to the SARS-CoV-2 virus. Our estimates of infections based on seroprevalence far exceed reported Coronavirus Disease 2019 (COVID-19) cases. Quality and standardized seroprevalence studies are essential to inform COVID-19 response, particularly in resource-limited regions. |
Link[5] Timeliness of reporting of SARS-CoV-2 seroprevalence results and their utility for infectious disease surveillance
Author: Claire Donnici, Natasha Ilincic, Christian Cao, Caseng Zhang, Gabriel Deveaux, David Clifton, David Buckeridge, Niklas Bobrovitz, Rahul K. Arora Publication date: 26 October 2022 Publication info: Epidemics, Volume 41, 2022, 100645, ISSN 1755-4365 Cited by: David Price 10:30 PM 27 November 2023 GMT Citerank: (3) 701020CANMOD – PublicationsPublications by CANMOD Members144B5ACA0, 704045Covid-19859FDEF6, 715376Serosurveillance859FDEF6 URL: DOI: https://doi.org/10.1016/j.epidem.2022.100645
| Excerpt / Summary [Epidemics, 26 October 2022]
Seroprevalence studies have been used throughout the COVID-19 pandemic to monitor infection and immunity. These studies are often reported in peer-reviewed journals, but the academic writing and publishing process can delay reporting and thereby public health action. Seroprevalence estimates have been reported faster in preprints and media, but with concerns about data quality. We aimed to (i) describe the timeliness of SARS-CoV-2 serosurveillance reporting by publication venue and study characteristics and (ii) identify relationships between timeliness, data validity, and representativeness to guide recommendations for serosurveillance efforts. We included seroprevalence studies published between January 1, 2020 and December 31, 2021 from the ongoing SeroTracker living systematic review. For each study, we calculated timeliness as the time elapsed between the end of sampling and the first public report. We evaluated data validity based on serological test performance and correction for sampling error, and representativeness based on the use of a representative sample frame and adequate sample coverage. We examined how timeliness varied with study characteristics, representativeness, and data validity using univariate and multivariate Cox regression. We analyzed 1844 studies. Median time to publication was 154 days (IQR 64–255), varying by publication venue (journal articles: 212 days, preprints: 101 days, institutional reports: 18 days, and media: 12 days). Multivariate analysis confirmed the relationship between timeliness and publication venue and showed that general population studies were published faster than special population or health care worker studies; there was no relationship between timeliness and study geographic scope, geographic region, representativeness, or serological test performance. Seroprevalence studies in peer-reviewed articles and preprints are published slowly, highlighting the limitations of using the academic literature to report seroprevalence during a health crisis. More timely reporting of seroprevalence estimates can improve their usefulness for surveillance, enabling more effective responses during health emergencies. |
Link[6] The evolution of SARS-CoV-2 seroprevalence in Canada: a time-series study, 2020–2023
Author: Tanya J. Murphy, Hanna Swail, Jaspreet Jain, David L. Buckeridge, et al. - Maureen Anderson, Philip Awadalla, Lesley Behl, Patrick E. Brown, Carmen L. Charlton, Karen Colwill, Steven J. Drews, Anne-Claude Gingras, Deena Hinshaw, Prabhat Jha, Jamil N. Kanji, Victoria A. Kirsh, Amanda L.S. Lang, Marc-André Langlois, Stephen Lee, Antoine Lewin, Sheila F. O’Brien, Chantale Pambrun, Kimberly Skead, David A. Stephens, Derek R. Stein, Graham Tipples, Paul G. Van Caeseele, Timothy G. Evans, Olivia Oxlade, Bruce D. Mazer Publication date: 14 August 2023 Publication info: CMAJ August 14, 2023 195 (31) E1030-E1037 Cited by: David Price 0:32 AM 28 November 2023 GMT Citerank: (3) 701020CANMOD – PublicationsPublications by CANMOD Members144B5ACA0, 704045Covid-19859FDEF6, 715376Serosurveillance859FDEF6 URL: DOI: https://doi.org/10.1503/cmaj.230249
| Excerpt / Summary [CMAJ, 14 August 2023]
Background: During the first year of the COVID-19 pandemic, the proportion of reported cases of COVID-19 among Canadians was under 6%. Although high vaccine coverage was achieved in Canada by fall 2021, the Omicron variant caused unprecedented numbers of infections, overwhelming testing capacity and making it difficult to quantify the trajectory of population immunity.
Methods: Using a time-series approach and data from more than 900 000 samples collected by 7 research studies collaborating with the COVID-19 Immunity Task Force (CITF), we estimated trends in SARS-CoV-2 seroprevalence owing to infection and vaccination for the Canadian population over 3 intervals: prevaccination (March to November 2020), vaccine roll-out (December 2020 to November 2021), and the arrival of the Omicron variant (December 2021 to March 2023). We also estimated seroprevalence by geographical region and age.
Results: By November 2021, 9.0% (95% credible interval [CrI] 7.3%–11%) of people in Canada had humoral immunity to SARS-CoV-2 from an infection. Seroprevalence increased rapidly after the arrival of the Omicron variant — by Mar. 15, 2023, 76% (95% CrI 74%–79%) of the population had detectable antibodies from infections. The rapid rise in infection-induced antibodies occurred across Canada and was most pronounced in younger age groups and in the Western provinces: Manitoba, Saskatchewan, Alberta and British Columbia.
Interpretation: Data up to March 2023 indicate that most people in Canada had acquired antibodies against SARS-CoV-2 through natural infection and vaccination. However, given variations in population seropositivity by age and geography, the potential for waning antibody levels, and new variants that may escape immunity, public health policy and clinical decisions should be tailored to local patterns of population immunity.
The COVID-19 pandemic defied expectations about immunity arising from infection and vaccination. During the first months of the pandemic, despite the burden on Canadian society and health systems, rates of symptomatic infection remained low, with 580 000 confirmed cases by December 2020, representing 1.6% of the Canadian population.1 Vaccines were widely distributed in Canada beginning in early 2021, with a rapid rise in vaccine coverage to 79% by fall of 2021,2 whereas cumulative reported cases of COVID-19 remained low, at 4.7% of the population.3 The arrival of Omicron variants and subvariants, however, caused an unprecedented increase in the number of infections. In short, the high vaccine coverage, combined with population immunity from infections in earlier waves of the pandemic, were insufficient to slow the spread of the Omicron variant.
Although the overall progression of confirmed cases and vaccination is clear, the underlying dynamics of population seropositivity are less obvious, yet critically important for policy and clinical decisions about vaccination and other preventive measures. A count of confirmed cases of COVID-19 is of limited use for understanding the evolution of population immunity because case ascertainment is biased by multiple factors. Most notably, access to laboratory-based polymerase chain reaction (PCR) testing varied across the country and, in many locations, was overwhelmed by demand after December 2021. In this context, serological surveillance provides an informative adjunct to monitoring confirmed cases, as seroprevalence offers a more direct measure of population humoral immunity.
We sought to describe the trajectory of SARS-CoV-2 seroprevalence in the Canadian population, as measured by anti-nucleocapsid (anti-N) and anti-spike protein (anti-S) antibody levels over 3 intervals: prevaccination (March to November 2020), vaccine roll-out (December 2020 to November 2021), and the Omicron variant waves (December 2021 to March 2023). We draw on seroprevalence estimates from multiple studies collaborating with the COVID-19 Immunity Task Force (CITF).4 In addition to describing the temporal evolution of population seropositivity in Canada, we highlight trends in infection-acquired and vaccine-induced seroprevalence by Canadian region and age.
|
|
|