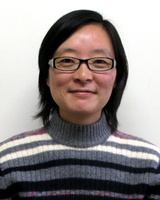
Research Interests
In general, I am interested in developing statistical models and methods motivated from scientific applications. In particular, I am currently working on the following areas.
(1) Interactions in regression models.
How to estimate and interpret interactions has been discussed in many research studies, such as epidemiological studies, environmental studies, social sciences, etc. We refer to interaction as departure from additivity, that is, the difference between the joint effect of all variables and the sum of their independent effects (see here for more information). It is challenging to model interactions when a large number of explanatory variables are involved in a study. So what happens if the interactions are misspecified? Interestingly, under some specific situations, the impact is not too bad (see here for details). More general investigations are needed in future.
(2) Errors-in-variables models.
It is a common difficulty in practice that some explanatory variables (predictors) cannot be precisely measured. This problem is often referred to as errors in variables. More specifically some literatures refer to measurement error for a continuous variable and misclassification for a categorical variable. It is well known that simply ignoring errors-in-variables leads to biased estimate. For a nice summary on this topic in linear regression context, please see Fuller (1987); for nonlinear models see Carroll, Ruppert, Stefanski and Crainiceanu (2006) . Currently I am involved in the following projects:
- Bayesian adjustment of misclassification in matched case-control studies;
- measurement error models in survival analysis;
- measurement error models in longitudinal data analysis.
(3) Markov chain Monte Carlo (MCMC) methodology.
MCMC methods, especially the Metropolis-Hastings algorithm (Metropolis et al., 1953; Hastings, 1970) and the Gibbs sampler (Geman and Geman, 1984; Gelfand and Smith, 1990) have been recognized as popular tools for the analysis of complex statistical models. For a comprehensive discussion of MCMC methods, please see Robert and Casella, Monte Carlo Statistical Methods, 2004. Currently I am interested in the following topics:
- developing more efficient MCMC algorithm (for some previous work see Chapter 4 in my PhD thesis);
- developing better diagnostic tools for MCMC convergence problem (for a good summary please see Cowles and Carlin, 1996, JASA, Vol 91, 1996).
Besides this, I am also interested in causal effect analysis motivated from epidemiological studies, spatial statistics problems motivated from remote sensing, and stochastic process modeling with application to genetics, biology, epidemiology, etc.