|
Charting a Future Action1 #714608 Charting a Future for Emerging Infectious Disease Modelling in Canada – April 2023 [1] | 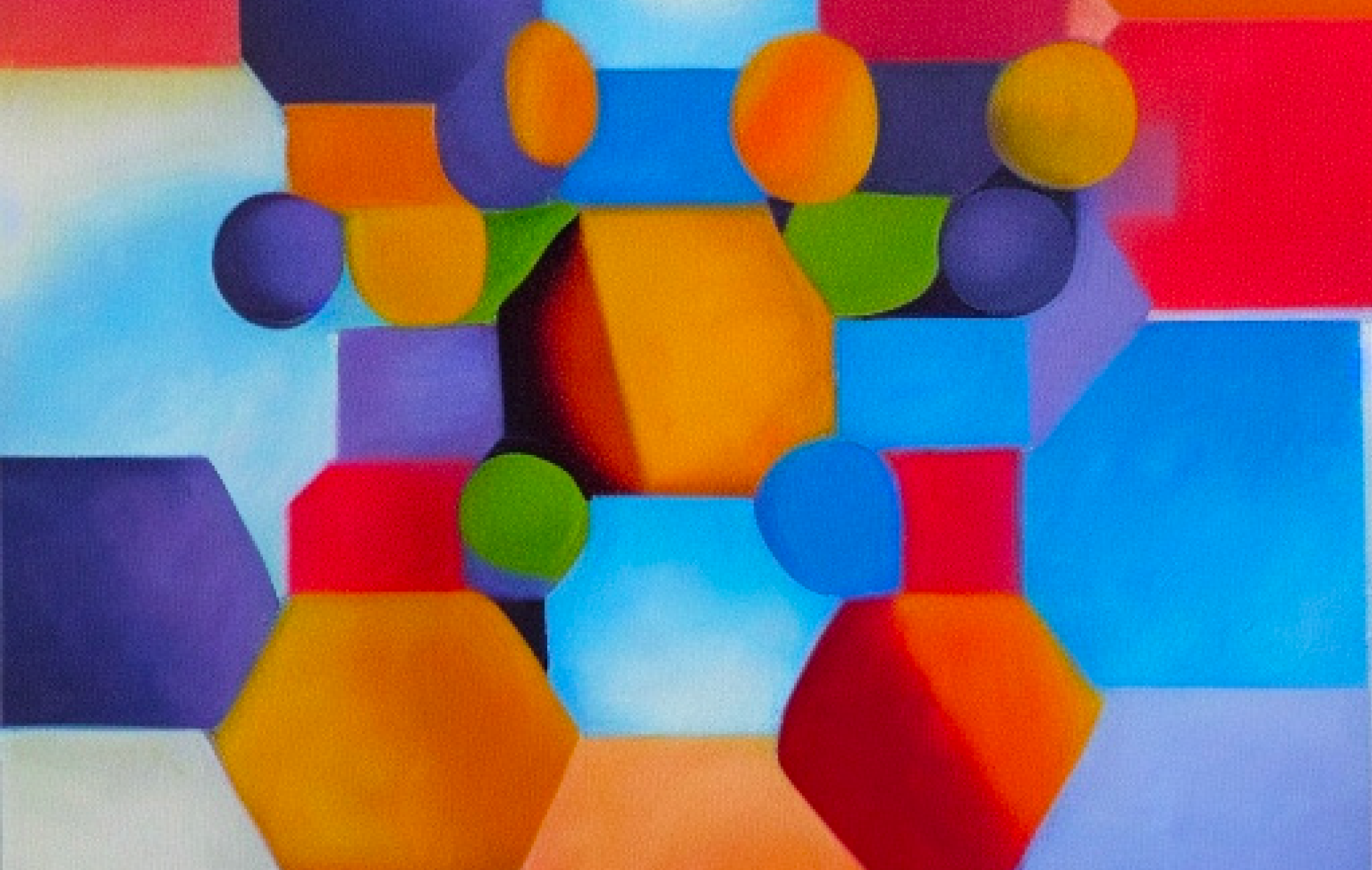
Executive SummaryWe propose an independent institute of emerging infectious disease modellers and policy experts, with an academic core, capable of renewing itself as needed. This institute will combine science and knowledge translation to inform decision-makers at all levels of government and ensure the highest level of preparedness (and readiness) for the next public health emergency. [1] The Public Health Modelling Institute will provide cost-effective, science-based modelling for public policymakers in an easily visualizable, integrated framework, which can respond in an agile manner to changing needs, questions, and data. To be effective, the Institute must link to modelling groups within government, who are best able to pose questions and convey results for use by public policymakers. 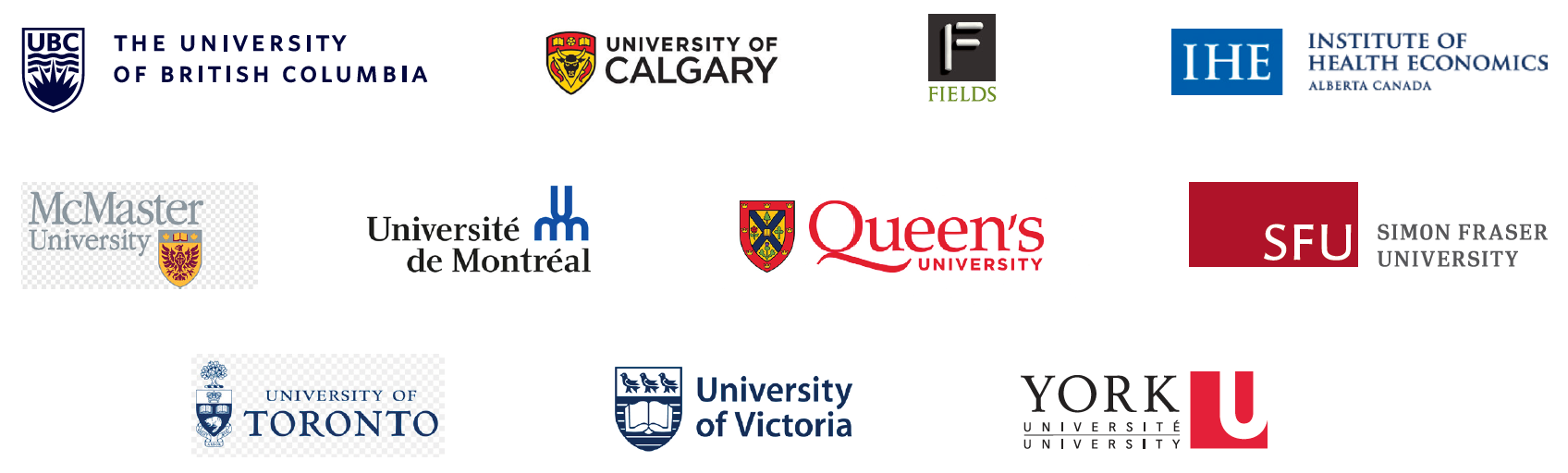
Quantitative models form a flexible suite of methods that can synthesize information from diverse fields, present this information intuitively and visually, support evidence-based policy, aid in communicating with the public, and quantify and manage uncertainty. Models can provide projections and scenarios and can support operational planning across multiple domains. The recent Emerging Infectious Disease Modelling (EIDM) program, created jointly by the Public Health Agency of Canada (PHAC) and Natural Sciences and Engineering Research Council of Canada (NSERC) in 2021, brought together over 100 modellers and other investigators from coast to coast. In collaboration with public health agencies (federal, provincial, and territorial), it fostered partnerships, collaboration, and trust across academic teams, government organizations, and public health institutions. It trained a high-quality workforce, and created state-of-the-art datasets and an arsenal of applied, interpretable models to support decision-making during the pandemic and beyond. Recent experience from the pandemic underscores the need for a lasting capacity for integrated, multi-disciplinary, whole-of-society analysis that can be rapidly deployed in an emergency, with sustained and direct linkages between infectious disease research, modelling, public health, evolution, economics, socio-economics, and policymaking. We need to ensure that there is a robust, trusted platform for rapid synthesis and consensus, spanning multiple domains and scales, ready to support decision-makers and decision-making. We envision a permanent institute to provide this capacity. In coordination with federal, provincial, and territorial public health agencies and Indigenous groups, it will: - undertake and harmonize research in this space across institutions,
- provide funding for continued modelling and capacity-building across a broad range of expertise, and
- ensure that Canada can better deal with future crises.
The institute will be an independent, apolitical authority that can help to formulate consensus “serviceable truths” in an emergency, rapidly bringing researchers together to work on policy activities when the next crisis does occur. Its core functions will also support robust pathogen surveillance, data access, and real-time analysis, and train a high-quality workforce in the requisite scientific, communication and collaboration skills. The Institute will connect a broad group of researchers on an ongoing basis. Importantly, its success will build upon the current bridges that have been developed between academic researchers and modellers, and those within public health agencies (federal, provincial, and territorial), who are best able to inform research directions and relay key messages to policy-makers. The Institute will have a permanent team of administrative staff, including an executive director, knowledge translation experts, and others. Together, these groups of people will form the core of long-lasting strategic relationships between the academic community and the policy advisors. The Institute will include mechanisms for compensating researchers (or their home institutions) when they are more intensively involved, for example as director, or as advisors in times of emergency. Formation of the Institute is a crucial step towards providing cost-effective, science-based modelling that can respond to changing needs, questions and data and ensuring the highest level of preparedness for the next public health emergency. IntroductionAs the world emerges from its most severe pandemic in over one hundred years, it is important to reflect on what happened so that we can better plan for the future. The crisis of COVID-19 galvanised a broad group of epidemiological modellers from academia who interacted with the Public Health Agency of Canada and provincial health authorities across Canada to help make informed decisions. The successes that were achieved are a testament to the enormous effort and dedication shown by individuals to build the required infrastructure and relationships for effective modelling and advising. This infrastructure, consisting of research groups with the relevant scientific expertise and those skilled in knowledge transfer, needs to be maintained and strengthened so that it can be readily deployed when new crises arise. Decisions during pandemics always require some form of modelling to integrate diverse data into an interpretable framework, to compare possible scenarios for trajectories over time, to evaluate interventions, and to estimate the likelihood of different outcomes and their associated costs and benefits. While models can be implicit, based on intuition or experience, the large number of factors at play make outcomes difficult to predict without explicitly incorporating key factors into a clear quantitative, scientific framework that can be used to make projections. Explicit quantitative models have been shown to be particularly useful when outcomes are unintuitive and policy options are not clear (e.g., informing vaccine rollout strategies (Aruffo et al. 2022a; Aruffo et al. 2022b), stay-at-home policy (Yuan et al. 2022b), school and community reopening (Yuan et al. 2022a), synergistic roles of different interventions (Tang et al., 2020), impact of public health interventions on different age groups and settings (McCarthy et al., 2020) or predicting future healthcare burdens (Tuite et al. 2020; Anderson et al. 2020; Zimmerman et al. 2021; Mishra et al. 2020)). In these cases, modellers, interacting with the broader scientific community, have a history of providing a “serviceable truth” (Jasanoff 2015), bringing together information from fields as disparate as evolutionary biology, immunology, epidemiology, and economics to formulate a scientific consensus that can help to provide a transparent and effective foundation for decision-making. The world was caught unprepared for COVID-19 modelling, with insufficient initial capacity for connecting modelling, the broader scientific community, and public health policy. Despite this slow start, the Canadian infectious disease modelling community has had many successes during the COVID-19 pandemic. These include the establishment of the first national COVID-19 modeling rapid response task force. In concert with the PHAC External Expert Modelling Group this task force informed and enabled PPE procurement and pharmaceutical purchasing needs (Betti et al. 2021b), predicting when Alpha, Gamma and Delta variants would begin to drive rising case numbers (BC COVID-19 Modelling Group 2021; Public Health Agency of Canada 2022), quantifying the conditions on public health measures to mitigate a pandemic wave (McCarthy et al. 2020; Tang et al. 2020), aiding the design of effective vaccine rollouts (Mulberry et al. 2021; Aruffo et al. 2022b; Betti et al. 2021a), defining and communicating the benefits of “flattening the curve” (Jackson 2020), and informing cost-effectiveness of policies with long- and short-term impacts (Cotton et al. 2022; Feng et al. 2022). Canadian modellers have built many new links, for example with the COVID-19 Genomics UK Consortium (https://www.cogconsortium.uk/), the Canadian COVID-19 Genomics Network (https://genomecanada.ca/challenge-areas/cancogen/), the Coronavirus Variants Rapid Response Network (https://covarrnet.ca/), and the COVID-19 Immunity Taskforce (https://www.covid19immunitytaskforce.ca/). Importantly, they have developed collaborative links with all of the provinces (see, for example, ON Science and Modelling tables (Hillmer et al. 2021 and https://covid19-sciencetable.ca/)) and some of the territories. Models have been incorporated explicitly into provincial analyses of COVID-19 (see, for example, Government of Manitoba 2022; Government of Alberta 2021; Government of Nova Scotia 2020; Government of Prince Edward Island 2020; Government of New Brunswick 2020; Brisson et al. 2022)). The PHAC convened weekly meetings of modellers throughout the country (the External Expert Modelling Group) to share methods, questions, results, and analyses; these meetings fostered networking in the Canadian modelling community from the start of the pandemic. These relationships provided a starting point for another success in building capacity in Canada: the Emerging Infectious Disease Modelling (EIDM) program. This joint venture between PHAC and the Natural Sciences and Engineering Research Council of Canada (NSERC) provided short-term funding to five key networks focused on public health, mathematics, statistics, One Health (human-wildlife-domestic animal-environment), evolution, and economic modelling, as well as the interactions among these themes. However, the EIDM funding is very short-term. Maintaining the strengths that have been developed requires a well-articulated long-term vision for emerging infectious disease modelling in Canada that builds on current successes and relationships. The goal of this paper [1] is to use our past experiences and connections to propose a long-term vision for connecting quantitative scientists and public health bodies in Canada. |
+Citaten (26) - CitatenVoeg citaat toeList by: CiterankMapLink[1] Charting a future for emerging infectious disease modelling in Canada
Citerend uit: Mark A. Lewis, Patrick Brown, Caroline Colijn, Laura Cowen, Christopher Cotton, Troy Day, Rob Deardon, David Earn, Deirdre Haskell, Jane Heffernan, Patrick Leighton, Kumar Murty, Sarah Otto, Ellen Rafferty, Carolyn Hughes Tuohy, Jianhong Wu, Huaiping Zhu Publication date: 26 April 2023 Geciteerd door: David Price 11:15 PM 2 November 2023 GMT
Citerank: (22) 679703EIDM?The Emerging Infectious Diseases Modelling Initiative (EIDM) – by the Public Health Agency of Canada and NSERC – aims to establish multi-disciplinary network(s) of specialists across the country in modelling infectious diseases to be applied to public needs associated with emerging infectious diseases and pandemics such as COVID-19. [1]7F1CEB7, 679761Caroline ColijnDr. Caroline Colijn works at the interface of mathematics, evolution, infection and public health, and leads the MAGPIE research group. She joined SFU's Mathematics Department in 2018 as a Canada 150 Research Chair in Mathematics for Infection, Evolution and Public Health. She has broad interests in applications of mathematics to questions in evolution and public health, and was a founding member of Imperial College London's Centre for the Mathematics of Precision Healthcare.10019D3ABAB, 679769Christopher CottonChristopher Cotton is a Professor of Economics at Queen’s University where he holds the Jarislowsky-Deutsch Chair in Economic & Financial Policy.10019D3ABAB, 679776David EarnProfessor of Mathematics and Faculty of Science Research Chair in Mathematical Epidemiology at McMaster University.10019D3ABAB, 679797Huaiping ZhuProfessor of mathematics at the Department of Mathematics and Statistics at York University, a York Research Chair (YRC Tier I) in Applied Mathematics, the Director of the Laboratory of Mathematical Parallel Systems at the York University (LAMPS), the Director of the Canadian Centre for Diseases Modelling (CCDM) and the Director of the One Health Modelling Network for Emerging Infections (OMNI-RÉUNIS). 10019D3ABAB, 679806Jane HeffernanJane Heffernan is a professor of infectious disease modelling in the Mathematics & Statistics Department at York University. She is a co-director of the Canadian Centre for Disease Modelling, and she leads national and international networks in mathematical immunology and the modelling of waning and boosting immunity.10019D3ABAB, 679812Jianhong WuProfessor Jianhong Wu is a University Distinguished Research Professor and Senior Canada Research Chair in industrial and applied mathematics at York University. He is also the NSERC Industrial Research Chair in vaccine mathematics, modelling, and manufacturing. 10019D3ABAB, 679826Laura CowenAssociate Professor in the Department of Mathematics and Statistics at the University of Victoria.10019D3ABAB, 679842Mark LewisProfessor Mark Lewis, Kennedy Chair in Mathematical Biology at the University of Victoria and Emeritus Professor at the University of Alberta.10019D3ABAB, 679858Patrick BrownAssociate Professor in the Centre for Global Health Research at St. Michael’s Hospital, and in the Department of Statistical Sciences at the University of Toronto.10019D3ABAB, 679859Patrick LeightonPatrick Leighton is a Professor of Epidemiology and Public Health at the Faculty of Veterinary Medicine, University of Montreal, and an active member of the Epidemiology of Zoonoses and Public Health Research Group (GREZOSP) and the Centre for Public Health Research (CReSP). 10019D3ABAB, 679869Rob DeardonAssociate Professor in the Department of Production Animal Health in the Faculty of Veterinary Medicine and the Department of Mathematics and Statistics in the Faculty of Science at the University of Calgary.10019D3ABAB, 679875Sarah OttoProfessor in Zoology. Theoretical biologist, Canada Research Chair in Theoretical and Experimental Evolution, and Killam Professor at the University of British Columbia.10019D3ABAB, 679890Troy DayTroy Day is a Professor and the Associate Head of the Department of Mathematics and Statistics at Queen’s University. He is an applied mathematician whose research focuses on dynamical systems, optimization, and game theory, applied to models of infectious disease dynamics and evolutionary biology.10019D3ABAB, 679893Kumar MurtyProfessor Kumar Murty is in the Department of Mathematics at the University of Toronto. His research fields are Analytic Number Theory, Algebraic Number Theory, Arithmetic Algebraic Geometry and Information Security. He is the founder of the GANITA lab, co-founder of Prata Technologies and PerfectCloud. His interest in mathematics ranges from the pure study of the subject to its applications in data and information security.10019D3ABAB, 686724Ellen RaffertyDr. Ellen Rafferty has a Master of Public Health and a PhD in epidemiology and health economics from the University of Saskatchewan. Dr. Rafferty’s research focuses on the epidemiologic and economic impact of public health policies, such as estimating the cost-effectiveness of immunization programs. She is interested in the incorporation of economics into immunization decision-making, and to that aim has worked with a variety of provincial and national organizations.10019D3ABAB, 701020CANMOD – PublicationsPublications by CANMOD Members144B5ACA0, 701037MfPH – Publications144B5ACA0, 701071OSN – Publications144B5ACA0, 701222OMNI – Publications144B5ACA0, 704045Covid-19859FDEF6, 715387SMMEID – Publications144B5ACA0 URL:
| Fragment- We propose an independent institute of emerging infectious disease modellers and policy experts, with an academic core, capable of renewing itself as needed. This institute will combine science and knowledge translation to inform decision-makers at all levels of government and ensure the highest level of preparedness (and readiness) for the next public health emergency. The Public Health Modelling Institute will provide cost-effective, science-based modelling for public policymakers in an easily visualizable, integrated framework, which can respond in an agile manner to changing needs, questions, and data. To be effective, the Institute must link to modelling groups within government, who are best able to pose questions and convey results for use by public policymakers. |
Link[2] Community structured model for vaccine strategies to control COVID19 spread: A mathematical study
Citerend uit: Elena Aruffo, Pei Yuan, Yi Tan, Evgenia Gatov, Effie Gournis, Sarah Collier, Nick Ogden, Jacques Bélair, Huaiping Zhu Publication date: 27 October 2022 Publication info: PLoS ONE 17(10): e0258648 Geciteerd door: David Price 2:03 PM 6 November 2023 GMT Citerank: (6) 679797Huaiping ZhuProfessor of mathematics at the Department of Mathematics and Statistics at York University, a York Research Chair (YRC Tier I) in Applied Mathematics, the Director of the Laboratory of Mathematical Parallel Systems at the York University (LAMPS), the Director of the Canadian Centre for Diseases Modelling (CCDM) and the Director of the One Health Modelling Network for Emerging Infections (OMNI-RÉUNIS). 10019D3ABAB, 679803Jacques BélairProfessor, Department of Mathematics and Statistics, Université de Montréal10019D3ABAB, 701037MfPH – Publications144B5ACA0, 704041Vaccination859FDEF6, 704045Covid-19859FDEF6, 715329Nick OgdenNicholas Ogden is a senior research scientist and Director of the Public Health Risk Sciences Division within the National Microbiology Laboratory at the Public Health Agency of Canada.10019D3ABAB URL: DOI: https://doi.org/10.1371/journal.pone.0258648
| Fragment- [PLoS ONE, 27 October 2022]
Initial efforts to mitigate the COVID-19 pandemic have relied heavily on non-pharmaceutical interventions (NPIs), including physical distancing, hand hygiene, and mask-wearing. However, an effective vaccine is essential to containing the spread of the virus. We developed a compartmental model to examine different vaccine strategies for controlling the spread of COVID-19. Our framework accounts for testing rates, test-turnaround times, and vaccination waning immunity. Using reported case data from the city of Toronto, Canada between Mar-Dec, 2020 we defined epidemic phases of infection using contact rates as well as the probability of transmission upon contact. We investigated the impact of vaccine distribution by comparing different permutations of waning immunity, vaccine coverage and efficacy throughout various stages of NPI’s relaxation in terms of cases and deaths. The basic reproduction number is also studied. We observed that widespread vaccine coverage substantially reduced the number of cases and deaths. Under phases with high transmission, an early or late reopening will result in new resurgence of the infection, even with the highest coverage. On the other hand, under phases with lower transmission, 60% of coverage is enough to prevent new infections. Our analysis of R0 showed that the basic reproduction number is reduced by decreasing the tests turnaround time and transmission in the household. While we found that household transmission can decrease following the introduction of a vaccine, public health efforts to reduce test turnaround times remain important for virus containment. |
Link[3] Mathematical modelling of vaccination rollout and NPIs lifting on COVID-19 transmission with VOC: a case study in Toronto, Canada
Citerend uit: Elena Aruffo, Pei Yuan, Yi Tan, Evgenia Gatov, Iain Moyles, Jacques Bélair, James Watmough, Sarah Collier, Julien Arino, Huaiping Zhu Publication date: 15 July 2022 Publication info: BMC Public Health, Volume 22, Article number: 1349 (2022) Geciteerd door: David Price 2:07 PM 6 November 2023 GMT
Citerank: (10) 679797Huaiping ZhuProfessor of mathematics at the Department of Mathematics and Statistics at York University, a York Research Chair (YRC Tier I) in Applied Mathematics, the Director of the Laboratory of Mathematical Parallel Systems at the York University (LAMPS), the Director of the Canadian Centre for Diseases Modelling (CCDM) and the Director of the One Health Modelling Network for Emerging Infections (OMNI-RÉUNIS). 10019D3ABAB, 679799Iain MoylesAssistant Professor in the Department of Mathematics and Statistics at York University. 10019D3ABAB, 679803Jacques BélairProfessor, Department of Mathematics and Statistics, Université de Montréal10019D3ABAB, 679805James WatmoughProfessor in the Department of Mathematics and Statistics at the University of New Brunswick.10019D3ABAB, 679817Julien ArinoProfessor and Faculty of Science Research Chair in Fundamental Science with the Department of Mathematics at the University of Manitoba.10019D3ABAB, 701037MfPH – Publications144B5ACA0, 701222OMNI – Publications144B5ACA0, 704041Vaccination859FDEF6, 704045Covid-19859FDEF6, 715328Nonpharmaceutical Interventions (NPIs)859FDEF6 URL: DOI: https://doi.org/10.1186/s12889-022-13597-9
| Fragment- [BMC Public Health, 15 July 2022]
Background: Since December 2020, public health agencies have implemented a variety of vaccination strategies to curb the spread of SARS-CoV-2, along with pre-existing Nonpharmaceutical Interventions (NPIs). Initial strategies focused on vaccinating the elderly to prevent hospitalizations and deaths, but with vaccines becoming available to the broader population, it became important to determine the optimal strategy to enable the safe lifting of NPIs while avoiding virus resurgence.
Methods: We extended the classic deterministic SIR compartmental disease-transmission model to simulate the lifting of NPIs under different vaccine rollout scenarios. Using case and vaccination data from Toronto, Canada between December 28, 2020, and May 19, 2021, we estimated transmission throughout past stages of NPI escalation/relaxation to compare the impact of lifting NPIs on different dates on cases, hospitalizations, and deaths, given varying degrees of vaccine coverages by 20-year age groups, accounting for waning immunity.
Results: We found that, once coverage among the elderly is high enough (80% with at least one dose), the main age groups to target are 20–39 and 40–59 years, wherein first-dose coverage of at least 70% by mid-June 2021 is needed to minimize the possibility of resurgence if NPIs are to be lifted in the summer. While a resurgence was observed for every scenario of NPI lifting, we also found that under an optimistic vaccination coverage (70% coverage by mid-June, along with postponing reopening from August 2021 to September 2021) can reduce case counts and severe outcomes by roughly 57% by December 31, 2021.
Conclusions: Our results suggest that focusing the vaccination strategy on the working-age population can curb the spread of SARS-CoV-2. However, even with high vaccination coverage in adults, increasing contacts and easing protective personal behaviours is not advisable since a resurgence is expected to occur, especially with an earlier reopening. |
Link[4] Efficacy of a “stay-at-home” policy on SARS-CoV-2 transmission in Toronto, Canada: a mathematical modelling study
Citerend uit: Pei Yuan, Juan Li, Elena Aruffo, Evgenia Gatov, Qi Li, Tingting Zheng, Nicholas H. Ogden, Beate Sander, Jane Heffernan, Sarah Collier, Yi Tan, Jun Li, Julien Arino, Jacques Bélair, James Watmough, Jude Dzevela Kong, Iain Moyles, Huaiping Zhu Publication date: 19 April 2022 Publication info: cmaj OPEN, April 19, 2022 10 (2) E367-E378 Geciteerd door: David Price 2:12 PM 6 November 2023 GMT
Citerank: (10) 679757Beate SanderCanada Research Chair in Economics of Infectious Diseases and Director, Health Modeling & Health Economics and Population Health Economics Research at THETA (Toronto Health Economics and Technology Assessment Collaborative).10019D3ABAB, 679797Huaiping ZhuProfessor of mathematics at the Department of Mathematics and Statistics at York University, a York Research Chair (YRC Tier I) in Applied Mathematics, the Director of the Laboratory of Mathematical Parallel Systems at the York University (LAMPS), the Director of the Canadian Centre for Diseases Modelling (CCDM) and the Director of the One Health Modelling Network for Emerging Infections (OMNI-RÉUNIS). 10019D3ABAB, 679799Iain MoylesAssistant Professor in the Department of Mathematics and Statistics at York University. 10019D3ABAB, 679805James WatmoughProfessor in the Department of Mathematics and Statistics at the University of New Brunswick.10019D3ABAB, 679806Jane HeffernanJane Heffernan is a professor of infectious disease modelling in the Mathematics & Statistics Department at York University. She is a co-director of the Canadian Centre for Disease Modelling, and she leads national and international networks in mathematical immunology and the modelling of waning and boosting immunity.10019D3ABAB, 679815Jude KongDr. Jude Dzevela Kong is an Assistant Professor in the Department of Mathematics and Statistics at York University and the founding Director of the Africa-Canada Artificial Intelligence and Data Innovation Consortium (ACADIC). 10019D3ABAB, 679817Julien ArinoProfessor and Faculty of Science Research Chair in Fundamental Science with the Department of Mathematics at the University of Manitoba.10019D3ABAB, 701222OMNI – Publications144B5ACA0, 715328Nonpharmaceutical Interventions (NPIs)859FDEF6, 715329Nick OgdenNicholas Ogden is a senior research scientist and Director of the Public Health Risk Sciences Division within the National Microbiology Laboratory at the Public Health Agency of Canada.10019D3ABAB URL: DOI: https://doi.org/10.9778/cmajo.20200242
| Fragment- Background: Globally, nonpharmaceutical interventions for COVID-19, including stay-at-home policies, limitations on gatherings and closure of public spaces, are being lifted. We explored the effect of lifting a stay-at-home policy on virus resurgence under different conditions.
Methods: Using confirmed case data from Toronto, Canada, between Feb. 24 and June 24, 2020, we ran a compartmental model with household structure to simulate the impact of the stay-at-home policy considering different levels of compliance. We estimated threshold values for the maximum number of contacts, probability of transmission and testing rates required for the safe reopening of the community.
Results: After the implementation of the stay-at-home policy, the contact rate outside the household fell by 39% (from 11.58 daily contacts to 7.11). The effective reproductive number decreased from 3.56 (95% confidence interval [CI] 3.02–4.14) on Mar. 12 to 0.84 (95% CI 0.79–0.89) on May 6. Strong adherence to stay-at-home policies appeared to prevent SARS-CoV-2 resurgence, but extending the duration of stay-at-home policies beyond 2 months had little added effect on cumulative cases (25 958 for 65 days of a stay-at-home policy and 23 461 for 95 days, by July 2, 2020) and deaths (1404 for 65 days and 1353 for 95 days). To avoid a resurgence, the average number of contacts per person per day should be kept below 9, with strict nonpharmaceutical interventions in place.
Interpretation: Our study demonstrates that the stay-at-home policy implemented in Toronto in March 2020 had a substantial impact on mitigating the spread of SARS-CoV-2. In the context of the early pandemic, before the emergence of variants of concern, reopening schools and workplaces was possible only with other nonpharmaceutical interventions in place.
Nonpharmaceutical interventions for COVID-19, including stay-at-home policies, isolation of cases and contact tracing, as well as physical distancing, handwashing and use of protective equipment such as face masks, are effective mitigation strategies for preventing virus spread.1–4 Many studies investigating SARS-CoV-2 transmission and nonpharmaceutical interventions point to the importance of within- and between-household transmission. 5–8 Although stay-at-home policies can help curb spread of SARS-CoV-2 in the community by reducing contacts outside the household,8 they can increase contacts among family members, leading to higher risk within the household, 9 with secondary infection rates in households shown to be as high as 30%–52.7%.5,10 Furthermore, prolonged periods of stay-at-home policies may not be practical because of the essential operations of society, and may directly or indirectly harm the economy and the physical and mental health of individuals.11,12 Therefore, it is important to assess the optimal length of policy implementation for preventing virus resurgence.
During the epidemic, stay-at-home policies have been used to mitigate virus spread. The proportion of people staying at home is a paramount factor for evaluating the effectiveness of this policy implementation. For example, symptomatic individuals, those who tested positive for SARS-CoV-2 infection, and traced contacts are more likely to remain in the home through self-isolation or quarantine than uninfected or asymptomatic individuals. 13 Hence, rates of testing, diagnosis, isolation of cases, contact tracing and quarantine of contacts, as well as public compliance with stay-at-home policies, are essential factors for determining virus transmission and the likelihood of epidemic resurgence after the lifting of restrictive closures.1 To allow for this level of complexity, we developed a household-based transmission model to capture differences in policy uptake behaviour using confirmed case data from Toronto, Canada.
Throughout the pandemic, Canadian provinces and territories have implemented restrictive closures of businesses, schools, workplaces and public spaces to reduce the number of contacts in the population and prevent further virus spread, with these restrictions lifted and reinstituted at various times.14 On Mar. 17, 2020, Ontario declared a state of emergency, with directives including stay-at-home policies.15
We aimed to evaluate the effect of the stay-at-home policy issued in March 2020 on the transmission of SARS-CoV-2 in Toronto, accounting for average household size, the degree of adherence to the stay-at-home policy, and the length of policy implementation. Additionally, on the basis of the average family size and local epidemic data, we estimated the basic reproduction number (R0) and effective reproduction number (Rt) and investigated potential thresholds for the number of contacts, testing rates and use of nonpharmaceutical interventions that would be optimal for mitigating the epidemic. Hence, we conducted simulations of dynamic population behaviour under different reopening and adherence scenarios, to compare different public health strategies in hopes of adding those evaluations to the scientific literature. |
Link[5] School and community reopening during the COVID-19 pandemic: a mathematical modelling study
Citerend uit: Pei Yuan, Elena Aruffo, Evgenia Gatov, Yi Tan, Qi Li, Nick Ogden, Sarah Collier, Bouchra Nasri, Iain Moyles, Huaiping Zhu Publication date: 2 February 2022 Publication info: R. Soc. open sci.9211883211883 Geciteerd door: David Price 2:13 PM 6 November 2023 GMT
Citerank: (9) 679759Bouchra NasriProfessor Nasri is a faculty member of Biostatistics in the Department of Social and Preventive Medicine at the University of Montreal. Prof. Nasri is an FRQS Junior 1 Scholar in Artificial Intelligence in Health and Digital Health. She holds an NSERC Discovery Grant in Statistics for time series dependence modelling for complex data.10019D3ABAB, 679797Huaiping ZhuProfessor of mathematics at the Department of Mathematics and Statistics at York University, a York Research Chair (YRC Tier I) in Applied Mathematics, the Director of the Laboratory of Mathematical Parallel Systems at the York University (LAMPS), the Director of the Canadian Centre for Diseases Modelling (CCDM) and the Director of the One Health Modelling Network for Emerging Infections (OMNI-RÉUNIS). 10019D3ABAB, 679799Iain MoylesAssistant Professor in the Department of Mathematics and Statistics at York University. 10019D3ABAB, 701037MfPH – Publications144B5ACA0, 701222OMNI – Publications144B5ACA0, 704045Covid-19859FDEF6, 715329Nick OgdenNicholas Ogden is a senior research scientist and Director of the Public Health Risk Sciences Division within the National Microbiology Laboratory at the Public Health Agency of Canada.10019D3ABAB, 715617Schools859FDEF6, 715617Schools859FDEF6 URL: DOI: https://doi.org/10.1098/rsos.211883
| Fragment- Operating schools safely during the COVID-19 pandemic requires a balance between health risks and the need for in-person learning. Using demographic and epidemiological data between 31 July and 23 November 2020 from Toronto, Canada, we developed a compartmental transmission model with age, household and setting structure to study the impact of schools reopening in September 2020. The model simulates transmission in the home, community and schools, accounting for differences in infectiousness between adults and children, and accounting for work-from-home and virtual learning. While we found a slight increase in infections among adults (2.2%) and children (4.5%) within the first eight weeks of school reopening, transmission in schools was not the key driver of the virus resurgence in autumn 2020. Rather, it was community spread that determined the outbreak trajectory, primarily due to increases in contact rates among adults in the community after school reopening. Analyses of cross-infection among households, communities and schools revealed that home transmission is crucial for epidemic progression and safely operating schools, while the degree of in-person attendance has a larger impact than other control measures in schools. This study suggests that safe school reopening requires the strict maintenance of public health measures in the community. |
Link[6] De-Escalation by Reversing the Escalation with a Stronger Synergistic Package of Contact Tracing, Quarantine, Isolation and Personal Protection: Feasibility of Preventing a COVID-19 Rebound in Ontario, Canada, as a Case Study
Citerend uit: Biao Tang, Francesca Scarabel, Nicola Luigi Bragazzi, Zachary McCarthy, Michael Glazer, Yanyu Xiao, Jane M. Heffernan, Ali Asgary, Nicholas Hume Ogden, Jianhong Wu Publication date: 16 May 2020 Publication info: Biology 2020, 9(5), 100, 16 May 2020 Geciteerd door: David Price 2:20 PM 6 November 2023 GMT Citerank: (1) 715294Contact tracing859FDEF6 URL: DOI: https://doi.org/10.3390/biology9050100
| Fragment- [Biology, 16 May 2020]
Since the beginning of the COVID-19 pandemic, most Canadian provinces have gone through four distinct phases of social distancing and enhanced testing. A transmission dynamics model fitted to the cumulative case time series data permits us to estimate the effectiveness of interventions implemented in terms of the contact rate, probability of transmission per contact, proportion of isolated contacts, and detection rate. This allows us to calculate the control reproduction number during different phases (which gradually decreased to less than one). From this, we derive the necessary conditions in terms of enhanced social distancing, personal protection, contact tracing, quarantine/isolation strength at each escalation phase for the disease control to avoid a rebound. From this, we quantify the conditions needed to prevent epidemic rebound during de-escalation by simply reversing the escalation process. |
Link[7] Quantifying the shift in social contact patterns in response to non-pharmaceutical interventions
Citerend uit: Zachary McCarthy, Yanyu Xiao, Francesca Scarabel, Biao Tang, Nicola Luigi Bragazzi, Kyeongah Nah, Jane M. Heffernan, Ali Asgary, V. Kumar Murty, Nicholas H. Ogden, Jianhong Wu Publication date: 1 December 2020 Publication info: Journal of Mathematics in Industry, Volume 10, Article number: 28 (2020) Geciteerd door: David Price 2:27 PM 6 November 2023 GMT
Citerank: (9) 679750Ali AsgaryAssociate Professor and Associate Director, Advanced Disaster, Emergency and Rapid Response Simulation (ADERSIM) in the School of Administrative Studies, and Adjunct Professor in the School of Information Technology, at York University.10019D3ABAB, 679806Jane HeffernanJane Heffernan is a professor of infectious disease modelling in the Mathematics & Statistics Department at York University. She is a co-director of the Canadian Centre for Disease Modelling, and she leads national and international networks in mathematical immunology and the modelling of waning and boosting immunity.10019D3ABAB, 679812Jianhong WuProfessor Jianhong Wu is a University Distinguished Research Professor and Senior Canada Research Chair in industrial and applied mathematics at York University. He is also the NSERC Industrial Research Chair in vaccine mathematics, modelling, and manufacturing. 10019D3ABAB, 679893Kumar MurtyProfessor Kumar Murty is in the Department of Mathematics at the University of Toronto. His research fields are Analytic Number Theory, Algebraic Number Theory, Arithmetic Algebraic Geometry and Information Security. He is the founder of the GANITA lab, co-founder of Prata Technologies and PerfectCloud. His interest in mathematics ranges from the pure study of the subject to its applications in data and information security.10019D3ABAB, 701037MfPH – Publications144B5ACA0, 704045Covid-19859FDEF6, 715328Nonpharmaceutical Interventions (NPIs)859FDEF6, 715329Nick OgdenNicholas Ogden is a senior research scientist and Director of the Public Health Risk Sciences Division within the National Microbiology Laboratory at the Public Health Agency of Canada.10019D3ABAB, 715617Schools859FDEF6 URL: DOI: https://doi.org/10.1186/s13362-020-00096-y
| Fragment- [Journal of Mathematics in Industry, 1 December 2020]
Social contact mixing plays a critical role in influencing the transmission routes of infectious diseases. Moreover, quantifying social contact mixing patterns and their variations in a rapidly evolving pandemic intervened by changing public health measures is key for retroactive evaluation and proactive assessment of the effectiveness of different age- and setting-specific interventions. Contact mixing patterns have been used to inform COVID-19 pandemic public health decision-making; but a rigorously justified methodology to identify setting-specific contact mixing patterns and their variations in a rapidly developing pandemic, which can be informed by readily available data, is in great demand and has not yet been established. Here we fill in this critical gap by developing and utilizing a novel methodology, integrating social contact patterns derived from empirical data with a disease transmission model, that enables the usage of age-stratified incidence data to infer age-specific susceptibility, daily contact mixing patterns in workplace, household, school and community settings; and transmission acquired in these settings under different physical distancing measures. We demonstrated the utility of this methodology by performing an analysis of the COVID-19 epidemic in Ontario, Canada. We quantified the age- and setting (household, workplace, community, and school)-specific mixing patterns and their evolution during the escalation of public health interventions in Ontario, Canada. We estimated a reduction in the average individual contact rate from 12.27 to 6.58 contacts per day, with an increase in household contacts, following the implementation of control measures. We also estimated increasing trends by age in both the susceptibility to infection by SARS-CoV-2 and the proportion of symptomatic individuals diagnosed. Inferring the age- and setting-specific social contact mixing and key age-stratified epidemiological parameters, in the presence of evolving control measures, is critical to inform decision- and policy-making for the current COVID-19 pandemic. |
Link[8] Mathematical modelling of COVID-19 transmission and mitigation strategies in the population of Ontario, Canada
Citerend uit: Ashleigh R. Tuite, David N. Fisman, Amy L. Greer Publication date: 11 May 2020 Publication info: CMAJ May 11, 2020 192 (19) E497-E505 Geciteerd door: David Price 2:32 PM 6 November 2023 GMT Citerank: (1) 715328Nonpharmaceutical Interventions (NPIs)859FDEF6 URL: DOI: https://doi.org/10.1503/cmaj.200476
| Fragment- [CMAJ, 11 May 2020]
BACKGROUND: Physical-distancing interventions are being used in Canada to slow the spread of severe acute respiratory syndrome coronavirus 2, but it is not clear how effective they will be. We evaluated how different nonpharmaceutical interventions could be used to control the coronavirus disease 2019 (COVID-19) pandemic and reduce the burden on the health care system.
METHODS: We used an age-structured compartmental model of COVID-19 transmission in the population of Ontario, Canada. We compared a base case with limited testing, isolation and quarantine to scenarios with the following: enhanced case finding, restrictive physical-distancing measures, or a combination of enhanced case finding and less restrictive physical distancing. Interventions were either implemented for fixed durations or dynamically cycled on and off, based on projected occupancy of intensive care unit (ICU) beds. We present medians and credible intervals from 100 replicates per scenario using a 2-year time horizon.
RESULTS: We estimated that 56% (95% credible interval 42%–63%) of the Ontario population would be infected over the course of the epidemic in the base case. At the epidemic peak, we projected 107 000 (95% credible interval 60 760–149 000) cases in hospital (non-ICU) and 55 500 (95% credible interval 32 700–75 200) cases in ICU. For fixed-duration scenarios, all interventions were projected to delay and reduce the height of the epidemic peak relative to the base case, with restrictive physical distancing estimated to have the greatest effect. Longer duration interventions were more effective. Dynamic interventions were projected to reduce the proportion of the population infected at the end of the 2-year period and could reduce the median number of cases in ICU below current estimates of Ontario’s ICU capacity.
INTERPRETATION: Without substantial physical distancing or a combination of moderate physical distancing with enhanced case finding, we project that ICU resources would be overwhelmed. Dynamic physical distancing could maintain health-system capacity and also allow periodic psychological and economic respite for populations. |
Link[9] Quantifying the impact of COVID-19 control measures using a Bayesian model of physical distancing
Citerend uit: Sean C. Anderson, Andrew M. Edwards, Madi Yerlanov, Nicola Mulberry, Jessica E. Stockdale, Sarafa A. Iyaniwura, Rebeca C. Falcao, Michael C. Otterstatter, Michael A. Irvine, Naveed Z. Janjua, Daniel Coombs, Caroline Colijn Publication date: 3 December 2020 Publication info: PLoS Comput Biol 16(12): e1008274 Geciteerd door: David Price 2:41 PM 6 November 2023 GMT Citerank: (2) 686691covidseircovidseir fits a Bayesian SEIR (Susceptible, Exposed, Infectious, Recovered) model to daily COVID-19 case data. The package focuses on estimating the fraction of the usual contact rate for individuals participating in physical distancing (social distancing). The model is coded in Stan. The model can accommodate multiple types of case data at once (e.g., reported cases, hospitalizations, ICU admissions) and accounts for delays between symptom onset and case appearance. [1]122C78CB7, 690180British Columbia COVID-19 GroupThe BC COVID-19 Modelling Group works on rapid response modelling of the COVID-19 pandemic, with a special focus on British Columbia and Canada.10015D3D3AB URL: DOI: https://doi.org/10.1371/journal.pcbi.1008274
| Fragment- Extensive non-pharmaceutical and physical distancing measures are currently the primary interventions against coronavirus disease 2019 (COVID-19) worldwide. It is therefore urgent to estimate the impact such measures are having. We introduce a Bayesian epidemiological model in which a proportion of individuals are willing and able to participate in distancing, with the timing of distancing measures informed by survey data on attitudes to distancing and COVID-19. We fit our model to reported COVID-19 cases in British Columbia (BC), Canada, and five other jurisdictions, using an observation model that accounts for both underestimation and the delay between symptom onset and reporting. We estimated the impact that physical distancing (social distancing) has had on the contact rate and examined the projected impact of relaxing distancing measures. We found that, as of April 11 2020, distancing had a strong impact in BC, consistent with declines in reported cases and in hospitalization and intensive care unit numbers; individuals practising physical distancing experienced approximately 0.22 (0.11–0.34 90% CI [credible interval]) of their normal contact rate. The threshold above which prevalence was expected to grow was 0.55. We define the “contact ratio” to be the ratio of the estimated contact rate to the threshold rate at which cases are expected to grow; we estimated this contact ratio to be 0.40 (0.19–0.60) in BC. We developed an R package ‘covidseir’ to make our model available, and used it to quantify the impact of distancing in five additional jurisdictions. As of May 7, 2020, we estimated that New Zealand was well below its threshold value (contact ratio of 0.22 [0.11–0.34]), New York (0.60 [0.43–0.74]), Washington (0.84 [0.79–0.90]) and Florida (0.86 [0.76–0.96]) were progressively closer to theirs yet still below, but California (1.15 [1.07–1.23]) was above its threshold overall, with cases still rising. Accordingly, we found that BC, New Zealand, and New York may have had more room to relax distancing measures than the other jurisdictions, though this would need to be done cautiously and with total case volumes in mind. Our projections indicate that intermittent distancing measures—if sufficiently strong and robustly followed—could control COVID-19 transmission. This approach provides a useful tool for jurisdictions to monitor and assess current levels of distancing relative to their threshold, which will continue to be essential through subsequent waves of this pandemic. |
Link[10] A queuing model for ventilator capacity management during the COVID-19 pandemic
Citerend uit: Samantha L. Zimmerman, Alexander R. Rutherford, Alexa van der Waall, Monica Norena, Peter Dodek Publication date: 23 May 2023 Publication info: Health Care Management Science, 22 May 2023, Volume 26, pages 200–216 (2023) Geciteerd door: David Price 2:48 PM 6 November 2023 GMT Citerank: (3) 679748Alexander RutherfordDr. Rutherford is the Director for the CSMG. Prior to joining the CSMG, he was the Scientific Executive Officer at the Pacific Institute for the Mathematical Sciences (PIMS). 10019D3ABAB, 701020CANMOD – PublicationsPublications by CANMOD Members144B5ACA0, 704045Covid-19859FDEF6 URL: DOI: https://doi.org/10.1007/s10729-023-09632-9
| Fragment- [Health Care Management Science, 22 May 2023]
We applied a queuing model to inform ventilator capacity planning during the first wave of the COVID-19 epidemic in the province of British Columbia (BC), Canada. The core of our framework is a multi-class Erlang loss model that represents ventilator use by both COVID-19 and non-COVID-19 patients. Input for the model includes COVID-19 case projections, and our analysis incorporates projections with different levels of transmission due to public health measures and social distancing. We incorporated data from the BC Intensive Care Unit Database to calibrate and validate the model. Using discrete event simulation, we projected ventilator access, including when capacity would be reached and how many patients would be unable to access a ventilator. Simulation results were compared with three numerical approximation methods, namely pointwise stationary approximation, modified offered load, and fixed point approximation. Using this comparison, we developed a hybrid optimization approach to efficiently identify required ventilator capacity to meet access targets. Model projections demonstrate that public health measures and social distancing potentially averted up to 50 deaths per day in BC, by ensuring that ventilator capacity was not reached during the first wave of COVID-19. Without these measures, an additional 173 ventilators would have been required to ensure that at least 95% of patients can access a ventilator immediately. Our model enables policy makers to estimate critical care utilization based on epidemic projections with different transmission levels, thereby providing a tool to quantify the interplay between public health measures, necessary critical care resources, and patient access indicators. |
Link[11] Estimated surge in hospital and intensive care admission because of the coronavirus disease 2019 pandemic in the Greater Toronto Area, Canada: a mathematical modelling study
Citerend uit: Sharmistha Mishra, Linwei Wang, Huiting Ma, Kirsty C Y Yiu, J. Michael Paterson, Eliane Kim, Michael J. Schull, Victoria Pequegnat, Anthea Lee, Lisa Ishiguro, Eric Coomes, Adrienne Chan, Mark Downing, David Landsman, Sharon Straus, Matthew Muller Publication date: 1 July 2020 Publication info: CMAJ Open, 01 Jul 2020, 8(3):E593-E604, PMCID: PMC7641231 Geciteerd door: David Price 3:04 PM 6 November 2023 GMT URL: DOI: https://doi.org/10.9778/cmajo.20200093 PMID: 32963024
| Fragment- Background: In pandemics, local hospitals need to anticipate a surge in health care needs. We examined the modelled surge because of the coronavirus disease 2019 (COVID-19) pandemic that was used to inform the early hospital-level response against cases as they transpired.
Methods: To estimate hospital-level surge in March and April 2020, we simulated a range of scenarios of severe acute respiratory syndrome coronavirus 2 (SARS-CoV-2) spread in the Greater Toronto Area (GTA), Canada, using the best available data at the time. We applied outputs to hospital-specific data to estimate surge over 6 weeks at 2 hospitals (St. Michael's Hospital and St. Joseph's Health Centre). We examined multiple scenarios, wherein the default (R0 = 2.4) resembled the early trajectory (to Mar. 25, 2020), and compared the default model projections with observed COVID-19 admissions in each hospital from Mar. 25 to May 6, 2020.
Results: For the hospitals to remain below non-ICU bed capacity, the default pessimistic scenario required a reduction in non-COVID-19 inpatient care by 38% and 28%, respectively, with St. Michael's Hospital requiring 40 new ICU beds and St. Joseph's Health Centre reducing its ICU beds for non-COVID-19 care by 6%. The absolute difference between default-projected and observed census of inpatients with COVID-19 at each hospital was less than 20 from Mar. 25 to Apr. 11; projected and observed cases diverged widely thereafter. Uncertainty in local epidemiological features was more influential than uncertainty in clinical severity.
Interpretation: Scenario-based analyses were reliable in estimating short-term cases, but would require frequent re-analyses. Distribution of the city's surge was expected to vary across hospitals, and community-level strategies were key to mitigating each hospital's surge. |
Link[12] Serviceable Truths: Science for Action in Law and Policy
Citerend uit: Sheila Jasanoff Publication date: 1 June 2015 Publication info: Texas Law Review, 93, 1723 Geciteerd door: David Price 3:17 PM 6 November 2023 GMT Citerank: (1) 714712H) Translating modelling into public health gainsEnsuring that emerging infectious disease modelling is translated into public health gains.8FFB597 URL:
| Fragment- [Texas Law Review, 93, 1723]
As the articles in this symposium issue attest, the relationship between law and science has begun to attract attention as an autonomous field of study, generating its own bodies of expertise and specialized scholarship. It is less obvious how the perspectives arising from within the community of legal practitioners and thinkers relate to a largely separate, but parallel, body of research and understanding from Science and Technology Studies (STS), a cross-disciplinary field that has for several decades been producing its own analyses of the relations between science, technology, and other authoritative institutions in society—including, of course, the law. Perhaps predictably, intersections between STS and legal studies have occurred most frequently around questions of evidence, since both fields share an interest in the nature and credibility of facts. Another area of topical convergence is intellectual property law, where authors may have dual training in law and STS. The shared interests of the two fields, however, bear on more fundamental questions of legal and political theory: questions about the nature of legitimacy and lawfulness in the modern world, where the actions of those in power must be held accountable to epistemic as well as normative standards—in short, to facts as well as to values. How to orchestrate that deeper engagement between STS and legal scholarship is one aim of this Article.
The road there can be charted in different ways. This symposium offers a pragmatic map. One can begin with cross-cutting topics at the intersections of science and law, especially criminal justice, bioethics, and the environment. In each of these areas, one approach is to pose questions aimed at improving the quality of scientific inputs to the legal process. Specifically, what evaluative standards should apply in conflicts over substance? Who should decide when experts disagree? And how should the results of knowledge processes be implemented? Under each of these headings, legal processes could benefit from a fuller grasp of relevant insights from STS, just as STS scholarship would gain depth and relevance by addressing more directly the kinds of issues and questions that seem most challenging from the standpoint of the law. In that sense, the pragmatic map may be as useful a starting point for future STS research as for legal studies.
This Article, however, departs from the topic–theme structure of the symposium to offer a more conceptual, indeed critical, perspective on law– science interactions. Here the concerns are not so much with making good decisions and hence with developing practical guidance on how the law should use or rely on scientific evidence and expert advice. Rather, the aim is to put society’s needs in the driver’s seat and explore how the two institutions could operate more effectively as partners in the central projects of governance in modern democracies: how to exercise power with reason, how to make good decisions in the face of epistemic as well as normative uncertainty, and how to strike an accountable balance between the sometimes conflicting pressures of knowledge and norms. In what follows, I sketch how STS understandings might help advance this kind of socially responsible collaboration between law and science… |
Link[13] COVID-19 Vaccination and Healthcare Demand
Citerend uit: Matthew I. Betti, Amira Hassan Abouleish, Victoria Spofford, Cory Peddigrew, Alan Diener, Jane M. Heffernan Publication date: 20 September 2021 Publication info: medRxiv 2021.09.15.21263628; Geciteerd door: David Price 6:27 PM 7 November 2023 GMT URL: DOI: https://doi.org/10.1101/2021.09.15.21263628
| Fragment- [medRxiv, 20 September 2021]
One of the driving concerns during any epidemic is the strain on the healthcare system. As we have seen many times over the globe with the COVID-19 pandemic, hospitals and ICUs can quickly become overwhelmed by cases. While strict periods of public health mitigation have certainly helped decrease incidence and thus healthcare demand, vaccination is the only clear long-term solution. In this paper, we develop a two-module model to forecast the effects of relaxation of non-pharmaceutical intervention and vaccine uptake on daily incidence, and the cascade effects on healthcare demand. The first module is a simple epidemiological model which incorporates non-pharmaceutical intervention, the relaxation of such measures and vaccination campaigns to predict caseloads into the the Fall of 2021. This module is then fed into a healthcare module which can forecast the number of doctor visits, the number of occupied hospital beds, number of occupied ICU beds and any excess demand of these. From this module we can also estimate the length of stay of individuals in ICU. For model verification and forecasting, we use the four most populous Canadian provinces as a case study. |
Link[14] Integrated vaccination and non-pharmaceutical interventions based strategies in Ontario, Canada, as a case study: a mathematical modelling study
Citerend uit: Matthew Betti, Nicola Luigi Bragazzi, Jane M. Heffernan, Jude Kong, Angie Raad Publication date: 14 July 2021 Publication info: J. R. Soc. Interface 18:20210009. Geciteerd door: David Price 6:35 PM 7 November 2023 GMT URL: DOI: https://doi.org/10.1098/rsif.2021.0009
| Fragment- [Journal of the Royal Society, Interface, 14 July 2021]
Recently, two coronavirus disease 2019 (COVID-19) vaccine products have been authorized in Canada. It is of crucial importance to model an integrated/combined package of non-pharmaceutical (physical/social distancing) and pharmaceutical (immunization) public health control measures. A modified epidemiological, compartmental SIR model was used and fit to the cumulative COVID-19 case data for the province of Ontario, Canada, from 8 September 2020 to 8 December 2020. Different vaccine roll-out strategies were simulated until 75% of the population was vaccinated, including a no-vaccination scenario. We compete these vaccination strategies with relaxation of non-pharmaceutical interventions. Non-pharmaceutical interventions were supposed to remain enforced and began to be relaxed on 31 January, 31 March or 1 May 2021. Based on projections from the data and long-term extrapolation of scenarios, relaxing the public health measures implemented by re-opening too early would cause any benefits of vaccination to be lost by increasing case numbers, increasing the effective reproduction number above 1 and thus increasing the risk of localized outbreaks. If relaxation is, instead, delayed and 75% of the Ontarian population gets vaccinated by the end of the year, re-opening can occur with very little risk. Relaxing non-pharmaceutical interventions by re-opening and vaccine deployment is a careful balancing act. Our combination of model projections from data and simulation of different strategies and scenarios, can equip local public health decision- and policy-makers with projections concerning the COVID-19 epidemiological trend, helping them in the decision-making process. |
Link[18] Quantifying the economic impacts of COVID-19 policy responses in (almost) real time
Citerend uit: Christopher Cotton, Bahman Kashi, Huw Lloyd-Ellis, Frederic Tremblay, Brett Crowley Publication date: 22 February 2022 Publication info: Canadian Journal of Economics/Revue canadienne d'économique, Volume 55, Issue S1 p. 406-445 Geciteerd door: David Price 7:10 PM 7 November 2023 GMT Citerank: (2) 701065OSN – Conceptual ApproachThe Network promises to build on the work done by the Royal Society of Canada (RSC) Task Force on COVID-19, Limestone Analytics, the Looking Glass Supercluster project, and the COVID Strategic Choices Group, to use an inter-sectoral approach to policy development and pandemic response.609FDDBE, 701071OSN – Publications144B5ACA0 URL: DOI: https://doi.org/10.1111/caje.12567
| Fragment- We develop a methodology to track and quantify the economic impacts of lockdown and reopening policies by Canadian provinces in response to the COVID-19 pandemic, using data that is available with a relatively short time lag. To do so, we adapt, calibrate and implement a dynamic, seasonally adjusted, input–output model with supply constraints. Our framework allows us to quantify potential scenarios that allow for dynamic complementarities between industries, seasonal fluctuations and changes in demand composition. Taking account of the observed variation in reopening strategies across provinces, we estimate the costs of the policy response in terms of lost hours of employment and production. Among other results, we show how a more aggressive response, even though it imposes higher economic costs in the short run, can lead to lower economic costs in the long run if it means avoiding future waves of lockdowns. |
Link[19] Epidemic Compartmental Models and Their Insurance Applications
Citerend uit: Runhuan Feng, José Garrido, Longhao Jin, Sooie-Hoe Loke, Linfeng Zhang Publication date: 24 October 2021 Publication info: Pandemics: Insurance and Social Protection, Chapter: Epidemic Compartmental Models and Their Insurance Applications, pp 13–40 Geciteerd door: David Price 7:16 PM 7 November 2023 GMT URL: DOI: https://doi.org/10.1007/978-3-030-78334-1_2
| Fragment- [Pandemics: Insurance and Social Protection, 24 October 2021]
Our society’s efforts to fight pandemics rely heavily on our ability to understand, model and predict the transmission dynamics of infectious diseases. Compartmental models are among the most commonly used mathematical tools to explain reported infections and deaths. This chapter offers a brief overview of basic compartmental models as well as several actuarial applications, ranging from product design and reserving of epidemic insurance, to the projection of healthcare demand and the allocation of scarce resources. The intent is to bridge classical epidemiological models with actuarial and financial applications that provide healthcare coverage and utilise limited healthcare resources during pandemics. |
Link[24] Ontario’s COVID-19 Modelling Consensus Table: mobilizing scientific expertise to support pandemic response
Citerend uit: Michael P. Hillmer, Patrick Feng, John R. McLaughlin, V. Kumar Murty, Beate Sander, Anna Greenberg, Adalsteinn D. Brown Publication date: 30 August 2021 Publication info: Canadian Journal of Public Health, Special Section on COVID-19: Innovations in Policy and Practice, 30 August 2021, Volume 112, pages799–806 (2021) Geciteerd door: David Price 7:33 PM 7 November 2023 GMT URL: DOI: https://doi.org/10.17269/s41997-021-00559-8
| Fragment- [Canadian Journal of Public Health, 30 August 2021]
Setting: COVID-19 has highlighted the need for credible epidemiological models to inform pandemic policy. Traditional mechanisms of commissioning research are ill-suited to guide policy during a rapidly evolving pandemic. At the same time, contracting with a single centre of expertise has been criticized for failing to reflect challenges inherent in specific modelling approaches.
Intervention: This report describes an alternative approach to mobilizing scientific expertise. Ontario’s COVID-19 Modelling Consensus Table (MCT) was created in March 2020 to enable rapid communication of credible estimates of the impact of COVID-19 and to accelerate learning on how the disease is spreading and what could slow its transmission. The MCT is a partnership between the province and academic modellers and consists of multiple groups of experts, health system leaders, and senior decision-makers. Armed with Ministry of Health data, the MCT meets once per week to share results from modelling exercises, generate consensus judgements of the likely future impact of COVID-19, and discuss decision-makers’ priorities.
Outcomes: The MCT has enabled swift access to data for participants, a structure for developing consensus estimates and communicating these to decision-makers, credible models to inform health system planning, and increased transparency in public reporting of COVID-19 data. It has also facilitated the rapid publication of research findings and its incorporation into government policy.
Implications: The MCT approach is one way to quickly draw on scientific advice outside of government and public health agencies. Beyond speed, this approach allows for nimbleness as experts from different organizations can be added as needed. It also shows how universities and research institutes have a role to play in crisis situations, and how this expertise can be marshalled to inform policy while respecting academic freedom and confidentiality. |
Link[26] Vaccine rollout strategies: The case for vaccinating essential workers early
Citerend uit: Nicola Mulberry, Paul Tupper, Erin Kirwin, Christopher McCabe, Caroline Colijn Publication date: 13 October 2021 Publication info: PLOS Glob Public Health 1(10): e0000020 Geciteerd door: David Price 5:10 PM 15 December 2023 GMT
Citerank: (11) 679761Caroline ColijnDr. Caroline Colijn works at the interface of mathematics, evolution, infection and public health, and leads the MAGPIE research group. She joined SFU's Mathematics Department in 2018 as a Canada 150 Research Chair in Mathematics for Infection, Evolution and Public Health. She has broad interests in applications of mathematics to questions in evolution and public health, and was a founding member of Imperial College London's Centre for the Mathematics of Precision Healthcare.10019D3ABAB, 679770Christopher McCabeDr. Christopher McCabe is the CEO and Executive Director of the Institute of Health Economics (IHE).10019D3ABAB, 679862Paul TupperProfessor in the Department of Mathematics at Simon Fraser University.10019D3ABAB, 685420Hospitals16289D5D4, 686720Erin KirwinErin Kirwin (she/her) is a Health Economist at the Institute of Health Economics (IHE) in Alberta, Canada. She holds a Bachelor of Arts (Honours) in Economics and International Development Studies from McGill University and a Master of Arts in Economics from the University of Alberta. Prior to joining the IHE, Erin was the Manager of Advanced Analytics at Alberta Health. Erin is a PhD candidate at the University of Manchester.10019D3ABAB, 701020CANMOD – PublicationsPublications by CANMOD Members144B5ACA0, 704041Vaccination859FDEF6, 704045Covid-19859FDEF6, 708794Health economics859FDEF6, 715454Workforce impact859FDEF6, 728545Long COVIDPost-acute sequelae of COVID-19 (PASC).859FDEF6 URL: DOI: https://doi.org/10.1371/journal.pgph.0000020
| Fragment- [PLOS Global Public Health, 13 October 2021]
In vaccination campaigns against COVID-19, many jurisdictions are using age-based rollout strategies, reflecting the much higher risk of severe outcomes of infection in older groups. In the wake of growing evidence that approved vaccines are effective at preventing not only adverse outcomes, but also infection, we show that such strategies are less effective than strategies that prioritize essential workers. This conclusion holds across numerous outcomes, including cases, hospitalizations, Long COVID (cases with symptoms lasting longer than 28 days), deaths and net monetary benefit. Our analysis holds in regions where the vaccine supply is limited, and rollout is prolonged for several months. In such a setting with a population of 5M, we estimate that vaccinating essential workers sooner prevents over 200,000 infections, over 600 deaths, and produces a net monetary benefit of over $500M. |
|
|