|
Dean Karlen Person1 #685229 R.M. Pearce Professor of Physics, University of Victoria and TRIUMF | 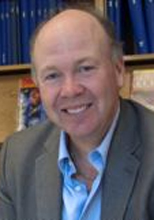
- Prof. Karlen has participated in particle physics experiments around the world. Beginning in the 1980s with the Mark-II experiment at SLAC, USA, and then in the 1990s with the OPAL experiment at CERN, Switzerland, he studied aspects of the standard model of particle physics with high energy electron-positron collisions. In the early 2000s, he helped lead the effort to develop and demonstrate the performance of a new type of precision detector, known as a micropattern Time Projection Chamber, for a future high energy linear electron-positron collider. He then led the project to incorporate this technology into the long baseline neutrino experiment, T2K in Japan, where three large volume detectors have been operating in the underground near detector facility since 2009. Prof. Karlen is the principle investigator for projects funded by the Canadian Foundation for Innovation and Provincial governments to build a superconducting electron accelerator and expand isotope production capabilities at TRIUMF, as part of the ARIEL project. From 2011-2017 he was director of the Victoria Subatomic Physics and Accelerator Research Centre (VISPA).
- Prior to coming to the University of Victoria and TRIUMF in 2002, Prof. Karlen was a professor in the Department of Physics at Carleton University, in Ottawa.
|
+Citaten (3) - CitatenVoeg citaat toeList by: CiterankMapLink[1] Characterizing the spread of CoViD-19
Citerend uit: Dean Karlen Publication date: 14 July 2020 Publication info: arXiv:2007.07156 Geciteerd door: David Price 2:27 PM 14 September 2022 GMT Citerank: (2) 685208python Population ModellerThe pyPM.ca software was developed to study and characterize the CoViD-19 epidemic.122C78CB7, 690180British Columbia COVID-19 GroupThe BC COVID-19 Modelling Group works on rapid response modelling of the COVID-19 pandemic, with a special focus on British Columbia and Canada.10015D3D3AB URL: DOI: https://doi.org/10.48550/arXiv.2007.07156
| Fragment- Since the beginning of the epidemic, daily reports of CoViD-19 cases, hospitalizations, and deaths from around the world have been publicly available. This paper describes methods to characterize broad features of the spread of the disease, with relatively long periods of constant transmission rates, using a new population modeling framework based on discrete-time difference equations. Comparative parameters are chosen for their weak dependence on model assumptions. Approaches for their point and interval estimation, accounting for additional sources of variance in the case data, are presented. These methods provide a basis to quantitatively assess the impact of changes to social distancing policies using publicly available data. As examples, data from Ontario and German states are analyzed using this framework. German case data show a small increase in transmission rates following the relaxation of lock-down rules on May 6, 2020. By combining case and death data from Germany, the mean and standard deviation of the time from infection to death are estimated. |
Link[3] Evaluation of the US COVID-19 Scenario Modeling Hub for informing pandemic response under uncertainty
Citerend uit: Emily Howerton, Lucie Contamin, Luke C. Mullany, et al. - Michelle Qin, Nicholas G. Reich, Samantha Bents, Rebecca K. Borchering, Sung-mok Jung, Sara L. Loo, Claire P. Smith, John Levander, Jessica Kerr, J. Espino, Willem G. van Panhuis, Harry Hochheiser, Marta Galanti, Teresa Yamana, Sen Pei, Jeffrey Shaman, Kaitlin Rainwater-Lovett, Matt Kinsey, Kate Tallaksen, Shelby Wilson, Lauren Shin, Joseph C. Lemaitre, Joshua Kaminsky, Juan Dent Hulse, Elizabeth C. Lee, Clifton D. McKee, Alison Hill, Dean Karlen, Matteo Chinazzi, Jessica T. Davis, Kunpeng Mu, Xinyue Xiong, Ana Pastore y Piontti, Alessandro Vespignani, Erik T. Rosenstrom, Julie S. Ivy, Maria E. Mayorga, Julie L. Swann, Guido España, Sean Cavany, Sean Moore, Alex Perkins, Thomas Hladish, Alexander Pillai, Kok Ben Toh, Ira Longini Jr., Shi Chen, Rajib Paul, Daniel Janies, Jean-Claude Thill, Anass Bouchnita, Kaiming Bi, Michael Lachmann, Spencer J. Fox, Lauren Ancel Meyers, Ajitesh Srivastava, Przemyslaw Porebski, Srini Venkatramanan, Aniruddha Adiga, Bryan Lewis, Brian Klahn, Joseph Outt Publication date: 20 November 2023 Publication info: Nature Communications, 14, Article number: 7260 (2023) Geciteerd door: David Price 5:23 PM 8 December 2023 GMT Citerank: (2) 701020CANMOD – PublicationsPublications by CANMOD Members144B5ACA0, 704045Covid-19859FDEF6 URL: DOI: https://doi.org/10.1038/s41467-023-42680-x
| Fragment- [Nature Communications, 20 November 2023]
Our ability to forecast epidemics far into the future is constrained by the many complexities of disease systems. Realistic longer-term projections may, however, be possible under well-defined scenarios that specify the future state of critical epidemic drivers. Since December 2020, the U.S. COVID-19 Scenario Modeling Hub (SMH) has convened multiple modeling teams to make months ahead projections of SARS-CoV-2 burden, totaling nearly 1.8 million national and state-level projections. Here, we find SMH performance varied widely as a function of both scenario validity and model calibration. We show scenarios remained close to reality for 22 weeks on average before the arrival of unanticipated SARS-CoV-2 variants invalidated key assumptions. An ensemble of participating models that preserved variation between models (using the linear opinion pool method) was consistently more reliable than any single model in periods of valid scenario assumptions, while projection interval coverage was near target levels. SMH projections were used to guide pandemic response, illustrating the value of collaborative hubs for longer-term scenario projections. |
|
|