|
Cindy Feng Person1 #679771 Associate Professor in the Department of Community Health and Epidemiology in the Faculty of Medicine at Dalhousie University. | 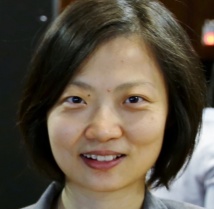
- Dr. Feng’s research interests lie primarily in developing biostatistical models for analyzing correlated data in which repeated measurements, hierarchical clustering, multiple outcome types, and spatially correlated data might occur. Dr. Feng's research has been funded by NSERC Discovery Grant, Canadian Statistical Sciences Institute and MITACS. She has a deep desire to bridge the gap between statistical methods and practice through pursuing methodological development and application of statistical methods in public health, which has led her to develop partnerships with many researchers from various disciplines, i.e. medicine, psychology, biology, and sociology.
Research Topics - Biostatistics
- Spatial statistics and disease mapping
- Statistical methods for survival and longitudinal data
- Environmental and ecological statistics
- Infectious disease surveillance
|
+Citavimą (2) - CitavimąPridėti citatąList by: CiterankMapLink[2] Predicting COVID-19 mortality risk in Toronto, Canada: a comparison of tree-based and regression-based machine learning methods
Cituoja: Cindy Feng, George Kephart, Elizabeth Juarez-Colunga Publication date: 27 November 2021 Publication info: BMC Med Res Methodol. 2021 Nov 27;21(1):267. Cituojamas: David Price 11:24 PM 17 November 2023 GMT Citerank: (1) 715387SMMEID – Publications144B5ACA0 URL: DOI: https://doi.org/10.1186/s12874-021-01441-4
| Ištrauka - [BMC Medical Research Methodology, 27 November 2021]
Background: Coronavirus disease (COVID-19) presents an unprecedented threat to global health worldwide. Accurately predicting the mortality risk among the infected individuals is crucial for prioritizing medical care and mitigating the healthcare system's burden. The present study aimed to assess the predictive accuracy of machine learning methods to predict the COVID-19 mortality risk.
Methods: We compared the performance of classification tree, random forest (RF), extreme gradient boosting (XGBoost), logistic regression, generalized additive model (GAM) and linear discriminant analysis (LDA) to predict the mortality risk among 49,216 COVID-19 positive cases in Toronto, Canada, reported from March 1 to December 10, 2020. We used repeated split-sample validation and k-steps-ahead forecasting validation. Predictive models were estimated using training samples, and predictive accuracy of the methods for the testing samples was assessed using the area under the receiver operating characteristic curve, Brier's score, calibration intercept and calibration slope.
Results: We found XGBoost is highly discriminative, with an AUC of 0.9669 and has superior performance over conventional tree-based methods, i.e., classification tree or RF methods for predicting COVID-19 mortality risk. Regression-based methods (logistic, GAM and LASSO) had comparable performance to the XGBoost with slightly lower AUCs and higher Brier's scores.
Conclusions: XGBoost offers superior performance over conventional tree-based methods and minor improvement over regression-based methods for predicting COVID-19 mortality risk in the study population. |
|
|