|
Lloyd T. Elliott Person1 #715254 Assistant Professor, Statistics and Actuarial Science at Simon Fraser University. | 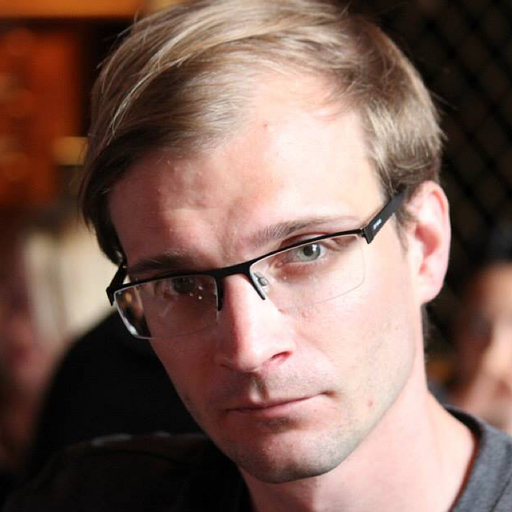
Areas of interest - Statistical genetics and genomics, bioinformatics; machine learning, Bayesian statistics, high-dimensional data.
|
+Citaten (6) - CitatenVoeg citaat toeList by: CiterankMapLink[3] Long time frames to detect the impact of changing COVID-19 control measures
Citerend uit: Jessica E Stockdale, Renny Doig, Joosung Min, Nicola Mulberry, Liangliang Wang, Lloyd T Elliott, Caroline Colijn Publication date: 16 June 2020 Publication info: medRxiv 2020.06.14.20131177 Geciteerd door: David Price 8:14 PM 16 November 2023 GMT Citerank: (1) 690180British Columbia COVID-19 GroupThe BC COVID-19 Modelling Group works on rapid response modelling of the COVID-19 pandemic, with a special focus on British Columbia and Canada.10015D3D3AB URL: DOI: https://doi.org/10.1101/2020.06.14.20131177
| Fragment- Background: Many countries have implemented population-wide interventions such as physical distancing measures, in efforts to control COVID-19. The extent and success of such measures has varied. Many jurisdictions with declines in reported COVID-19 cases are moving to relax measures, while others are continuing to intensify efforts to reduce transmission.
Aim: We aim to determine the time frame between a change in COVID-19 measures at the population level and the observable impact of such a change on cases.
Methods: We examine how long it takes for there to be a substantial difference between the cases that occur following a change in control measures and those that would have occurred at baseline. We then examine how long it takes to detect a difference, given delays and noise in reported cases. We use changes in population-level (e.g., distancing) control measures informed by data and estimates from British Columbia, Canada.
Results: We find that the time frames are long: it takes three weeks or more before we might expect a substantial difference in cases given a change in population-level COVID-19 control, and it takes slightly longer to detect the impacts of the change. The time frames are shorter (11-15 days) for dramatic changes in control, and they are impacted by noise and delays in the testing and reporting process, with delays reaching up to 25-40 days.
Conclusion: The time until a change in broad control measures has an observed impact is longer than is typically understood, and is longer than the mean incubation period (time between exposure than onset) and the often used 14 day time period. Policy makers and public health planners should consider this when assessing the impact of policy change, and efforts should be made to develop rapid, consistent real-time COVID-19 surveillance. |
Link[4] HostSeq: a Canadian whole genome sequencing and clinical data resource
Citerend uit: S Yoo, E Garg, LT Elliott, LJ Strug, et al. - RJ Hung, AR Halevy, JD Brooks, SB Bull, F Gagnon, CMT Greenwood, JF Lawless, AD Paterson, L Sun, MH Zawati, J Lerner-Ellis, RJS Abraham, I Birol, G Bourque, J-M Garant, C Gosselin, J Li, J Whitney, B Thiruvahindrapuram, J-A Herbrick, M Lorenti, MS Reuter, OO Adeoye, S Liu, U Allen, FP Bernier, CM Biggs, AM Cheung, J Cowan, M Herridge, DM Maslove, BP Modi, V Mooser, SK Morris, M Ostrowski, RS Parekh, G Pfeffer, O Suchowersky, J Taher, J Upton, RL Warren, RSM Yeung, N Aziz, SE Turvey, BM Knoppers, M Lathrop, SJM Jones, SW Scherer Publication date: 2 May 2023 Publication info: BMC Genomic Data volume 24, Article number: 26 (2023) Geciteerd door: David Price 8:26 PM 16 November 2023 GMT Citerank: (3) 701020CANMOD – PublicationsPublications by CANMOD Members144B5ACA0, 704045Covid-19859FDEF6, 708734Genomics859FDEF6 URL: DOI: https://doi.org/10.1186/s12863-023-01128-3
| Fragment- [BMC Genomic Data, 2 May 2023]
HostSeq was launched in April 2020 as a national initiative to integrate whole genome sequencing data from 10,000 Canadians infected with SARS-CoV-2 with clinical information related to their disease experience. The mandate of HostSeq is to support the Canadian and international research communities in their efforts to understand the risk factors for disease and associated health outcomes and support the development of interventions such as vaccines and therapeutics. HostSeq is a collaboration among 13 independent epidemiological studies of SARS-CoV-2 across five provinces in Canada. Aggregated data collected by HostSeq are made available to the public through two data portals: a phenotype portal showing summaries of major variables and their distributions, and a variant search portal enabling queries in a genomic region. Individual-level data is available to the global research community for health research through a Data Access Agreement and Data Access Compliance Office approval. Here we provide an overview of the collective project design along with summary level information for HostSeq. We highlight several statistical considerations for researchers using the HostSeq platform regarding data aggregation, sampling mechanism, covariate adjustment, and X chromosome analysis. In addition to serving as a rich data source, the diversity of study designs, sample sizes, and research objectives among the participating studies provides unique opportunities for the research community. |
Link[5] Under-reporting of COVID-19 in the Northern Health Authority region of British Columbia
Citerend uit: Matthew R. P. Parker, Yangming Li, Lloyd T. Elliott, Junling Ma, Laura L. E. Cowen Publication date: 1 November 2021 Publication info: Canadian Journal of Statistics, Volume 49, Issue 4 p. 1018-1038 Geciteerd door: David Price 8:29 PM 16 November 2023 GMT Citerank: (4) 679826Laura CowenAssociate Professor in the Department of Mathematics and Statistics at the University of Victoria.10019D3ABAB, 701037MfPH – Publications144B5ACA0, 704045Covid-19859FDEF6, 7147033e N-mixture (hidden Markov) type models123AECCD8 URL: DOI: https://doi.org/10.1002/cjs.11664
| Fragment- [Canadian Journal of Statistics, 1 November 2021]
Asymptomatic and pauci-symptomatic presentations of COVID-19 along with restrictive testing protocols result in undetected COVID-19 cases. Estimating undetected cases is crucial to understanding the true severity of the outbreak. We introduce a new hierarchical disease dynamics model based on the N-mixtures hidden population framework. The new models make use of three sets of disease count data per region: reported cases, recoveries and deaths. Treating the first two as under-counted through binomial thinning, we model the true population state at each time point by partitioning the diseased population into the active, recovered and died categories. Both domestic spread and imported cases are considered. These models are applied to estimate the level of under-reporting of COVID-19 in the Northern Health Authority region of British Columbia, Canada, during 30 weeks of the provincial recovery plan. Parameter covariates are easily implemented and used to improve model estimates. We compare two distinct methods of model-fitting for this case study: (1) maximum likelihood estimation, and (2) Bayesian Markov chain Monte Carlo. The two methods agreed exactly in their estimates of under-reporting rate. When accounting for changes in weekly testing volumes, we found under-reporting rates varying from 60.2% to 84.2%. |
Link[6] Estimation of SARS-CoV-2 antibody prevalence through integration of serology and incidence data
Citerend uit: Liangliang Wang, Joosung Min, Renny Doig, Lloyd T Elliott, Caroline Colijn Publication date: 28 March 2021 Publication info: medRxiv 2021.03.27.21254471 Geciteerd door: David Price 8:31 PM 16 November 2023 GMT Citerank: (2) 690180British Columbia COVID-19 GroupThe BC COVID-19 Modelling Group works on rapid response modelling of the COVID-19 pandemic, with a special focus on British Columbia and Canada.10015D3D3AB, 715376Serosurveillance859FDEF6 URL: DOI: https://doi.org/10.1101/2021.03.27.21254471
| Fragment- Serology tests for SARS-CoV-2 provide a paradigm for estimating the number of individuals who have had infection in the past (including cases that are not detected by routine testing, which has varied over the course of the pandemic and between jurisdictions). Classical statistical approaches to such estimation do not incorporate case counts over time, and may be inaccurate due to uncertainty about the sensitivity and specificity of the serology test. In this work, we provide a joint Bayesian model for case counts and serological data, integrating uncertainty through priors on the sensitivity and specificity. We also model the Phases of the pandemic with exponential growth and decay. This model improves upon maximum likelihood estimates by conditioning on more data, and by taking into account the epidemiological trajectory. We apply our model to the greater Vancouver area, British Columbia, Canada with data acquired during Phase 1 of the pandemic. |
|
|