|
Manos Papagelis Person1 #679837 I am an Associate Professor of Electrical Engineering and Computer Science (EECS) at the Lassonde School of Engineering, York University. I am faculty member of the Data Mining Lab. | 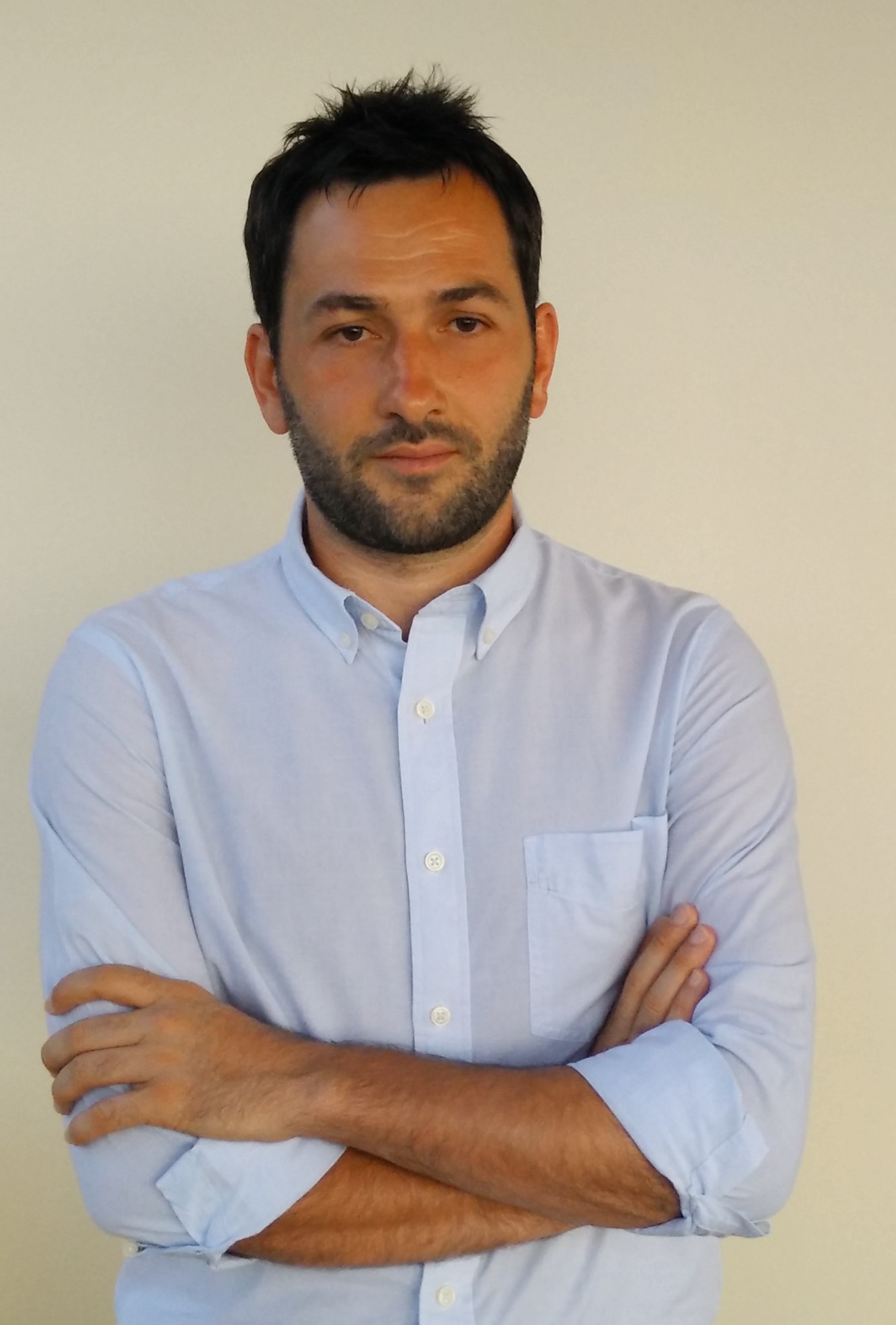
- Trajectory Data Mining: Advances in location acquisition and tracking devices have given rise to the generation of enormous trajectory data consisting of spatial and temporal information of moving objects, such as persons, vehicles or animals. The primary focus of our research is on discovery of network patterns and dynamics through mining trajectory data streams. This describes a special type of trajectory mining task that seeks to efficiently discover pair-wise relationships (interactions) among moving objects over time. Mining trajectory data streams to find interesting network patterns is of increased research interest due to a broad range of useful applications, including analysis of transportation systems and location-based social networks.
- Network Representation Learning: With a growing number of networks – social, technological, biological – becoming available and representing an ever increasing amount of information, the ability to easily and effectively perform large-scale network mining and analysis is key to revealing the underlying dynamics of these networks, not easily observable before. Traditional approaches to network mining and analysis inherit a number of limitations; typically algorithms don't scale well (due to ineffective representation of network data) and require domain-expertise. More recently and to address the aforementioned limitations, there is a fast-growing interest in learning low-dimensional and continuous representations of networks. Representing networks into low-dimensional spaces occurs in an agnostic way (without domain-expertise) and has the potential to improve the performance of many data mining tasks that now need to operate in lower dimensions. Network mining can support a variety of applications in diverse disciplines and has the potential to impact different industries.
- Streaming & Dynamic Graph Mining: Large-scale graph mining and analysis is key to revealing the underlying dynamics of networks, not easily observable before. The conventional computational approach for performing graph/network analysis assumes there is a static network topology (and/or data) that is provided as input to a graph algorithm, which (always) terminates (i.e., produces an outcome or fails). Analyzing massive graphs via classical algorithms casts its own unique challenges (e.g, memory/time overhead), but the conventional approach is mostly insufficient for many modern data processing needs. Over the last years, there has been considerable interest in designing algorithms for processing graphs in the data stream model, where the input is defined by a stream of graph data (e.g., a stream of edges), and the graph algorithm, aware of these changes, must be able to accept the changes faster than a naive re-computation of an algorithm on static graphs. Algorithms in this model must operate under specific constraints: (i) the input stream must be processed in the order it arrives, and (ii) the processing can only use a limited amount of memory.
|
+Citavimą (2) - CitavimąPridėti citatąList by: CiterankMapLink[2] Microscopic modeling of spatiotemporal epidemic dynamics
Cituoja: Tilemachos Pechlivanoglou, Gian Alix, Nina Yanin, Jing Li, Farzaneh Heidari, Manos Papagelis Publication date: November 2022 Publication info: SpatialEpi '22: Proceedings of the 3rd ACM SIGSPATIAL International Workshop on Spatial Computing for Epidemiology, November 2022, Pages 11–21 Cituojamas: David Price 2:39 PM 18 November 2023 GMT Citerank: (1) 701222OMNI – Publications144B5ACA0 URL: DOI: https://doi.org/10.1145/3557995.3566116
| Ištrauka - [Proceedings of the 3rd ACM SIGSPATIAL International Workshop on Spatial Computing for Epidemiology, November 2022]
Conventional techniques of epidemic modeling are based on compartmental models, where population groups are transitioning from one compartment to another - for example, S, I, or R, (Susceptible, Infectious, or Recovered). Then, they focus on learning macroscopic properties of disease spreading, such as the transition rates between compartments. Although these models are useful in studying epidemic dynamics, they lack the granularity needed for analyzing individual behaviors during an epidemic and understanding the relationship between individual decisions and the spread of the disease. In this paper, we develop microscopic models of spatiotemporal epidemic dynamics informed by mobility patterns of individuals and their interactions. In contrast to macroscopic models, microscopic epidemic models focus on individuals and their properties, such as their activity level, mobility behaviors, and impact of mobility behavior changes. Our microscopic spatiotemporal epidemic model allows to (i) assess the risk of infection of an individual based on mobility patterns;(ii) assess the risk of infection associated with specific geographic areas and points-of-interest (POIs);(iii) assess the risk of infection of a trip in an urban environment;(iv) provide trip recommendation for mitigating the risk of infection;and (v) assess targeted intervention strategies that aim to control the epidemic spreading. Our work provides an evidence-based data-driven model to inform individuals about the infection risks associated with their mobility behavior during a pandemic, providing at the same time safer alternatives. It can also inform public policy about the effectiveness of targeted intervention strategies that aim to contain or mitigate the epidemic spread compared to horizontal measures. |
|
|