|
CANMOD – People Person1 #679712 CANMOD is a national network, with members located across the country and associated with a broader Emerging Infectious Disease Modelling (EIDM) initiative. We are a community of modellers, statisticians, epidemiologists, public health decision-makers, and those implementing and delivering interventions. | 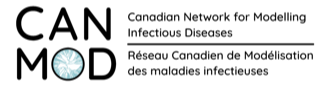
Tags: CANMOD Network |
+Αναφορές (1) - ΑναφορέςΠροσθήκη αναφοράςList by: CiterankMapLink[1] NSERC Funding Decisions: Emerging Infectious Diseases Modelling Initiative
Συγγραφέας: NSERC Παρατέθηκε από: David Price 11:25 AM 7 June 2021 GMT
Citerank: (9) 679703EIDM?The Emerging Infectious Diseases Modelling Initiative (EIDM) – by the Public Health Agency of Canada and NSERC – aims to establish multi-disciplinary network(s) of specialists across the country in modelling infectious diseases to be applied to public needs associated with emerging infectious diseases and pandemics such as COVID-19. [1]7F1CEB7, 679714OMNI – PeopleOur English and French acronyms of the network, One Health Modelling Network for Emerging Infections (OMNI)/RÉseau UNe seule santé sur la modélisation des InfectionS (RÉUNIS) symbolize universality and the bringing together of people and ideas. This is precisely what we have done, having amassed an amazing network of interdisciplinary people with a commitment to a One Health approach to stopping emerging infectious diseases (EIDs) at all levels.10019D3ABAB, 679715OSN – PeopleThe One Society Network (OSN), led by Dr. Christopher McCabe at the University of Alberta, will include developing modelling for evaluating alternative policy responses during pandemics for all sectors of the economy and aspects of society, including marginalised groups. They will also be collaborating on multi-disciplinary training programs for skills development to support public policy making in future pandemics. [1]10019D3ABAB, 679716SMMEID – PeopleStatistical Methods for Managing Emerging Infectious Diseases (SMMEID), led by Dr. Patrick Brown at the University of Toronto, will develop methods and tools to get an accurate picture of the nature and extent of infectious disease transmission in the population, relying on real-world data from administrative sources and surveys. They are seeking to augment Canada's capacity to respond to emerging infectious diseases. [1]10019D3ABAB, 701002OMNIThe One Health Modelling Network for Emerging Infections (OMNI), led by Dr. Huaiping Zhu at York University, will identify gaps that can be used to prioritize more targeted surveillance or data collection and then use those data to refine models. This work will contribute to an improved understanding of the conditions that enable pathogen spread and transmission and identify actions that can most effectively manage these conditions. [1]1002079B9B9, 701005CANMOD?CANadian Network for MODelling infectious Disease / Réseau CANadien de MODélisation des maladies infectieuses1002079B9B9, 701006MfPHMathematics for Public Health (MfPH), led by Dr. V. Kumar Murty, Director of the Fields Institute and Professor at the University of Toronto, will aim to bridge the gap between mathematical research and real public health issues. The team will seek to produce models that are effective, practical and reliable for applications to public health issues for COVID-19 as well as boost Canada’s future pandemic preparedness. [1]1002079B9B9, 701007OSNThe One Society Network (OSN), led by Dr. Christopher McCabe at the University of Alberta, will include developing modelling for evaluating alternative policy responses during pandemics for all sectors of the economy and aspects of society, including marginalised groups. They will also be collaborating on multi-disciplinary training programs for skills development to support public policy making in future pandemics. [1]1002079B9B9, 701008SMMEIDThis project assembles the top biostatisticians in Canada working on infectious diseases, and joins them with epidemiologists developing novel methods for data collection during the COVID-19 pandemic. Our group is developing methods and tools to get an accurate picture of the nature and extent of infectious disease transmission in the population, relying on real-world data from administrative sources and surveys. [2]1002079B9B9 URL:
|
|
|