Venkata Duvvuri
Dr. Duvvuri is an Assistant Professor and leads an interdisciplinary research program advancing genomic surveillance research, development, and practice in the Department of Laboratory Medicine & Pathobiology at the University of Toronto. He is also a Scientist in Machine Learning at Public Health Ontario.
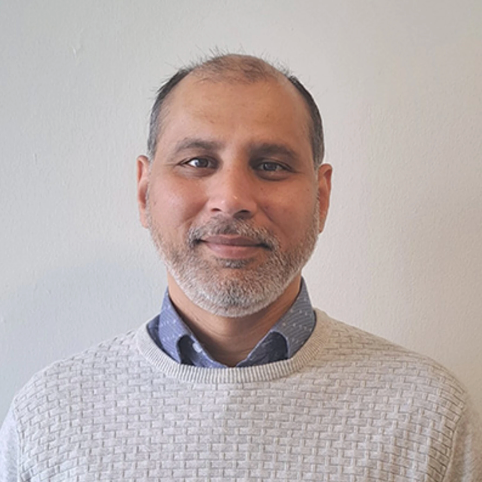
Areas of Expertise
- data science and machine learning
- genomic epidemiology
- public health microbiology
- one health approach
- health administrative and Electronic Health Record (EHR) data
Appointments
Assistant Professor, Laboratory Medicine and Pathobiology, University of Toronto
Academic Degrees and Accreditations
- Postgraduate program in Artificial Intelligence and Machine Learning: Business Analytics, McCombs School of Business, The University of Texas at Austin
- Master of Public Health, School of Public Health and Health Systems, University of Waterloo
- Master of Science (Biology), Faculty of Science, York University
- Doctor of Philosophy (Zoology), Osmania University
PHO Research Interests
- Incorporate data science and machine learning into public health microbiology and genomics to develop risk discovery, assessment and prediction models
- Develop combined genomic and epidemiological methods and approaches to study pathogen transmission and epidemic dynamics
- Identify genomic biomarkers from pathogen genomes to develop novel and rapid molecular diagnostic tests and vaccine candidates using immunoinformatics
- Accelerate public health surveillance and planning by integrating public health and healthcare strategies
PHO Research Activities
- Develop a genome-based machine learning model to address challenges of antimicrobial resistance (AMR) in public health
- Examine the evolutionary and transmission dynamics of viral (for example COVID-19, Respiratory syncytial virus (RSV)), bacterial and fungal pathogens using phylodynamics
- Collaborate on the development of novel and rapid diagnostics for infectious diseases using immunoinformatics
- Provide expertise in data science protocols and machine learning approaches